Find and fix model errors (error analysis)
How to perform model error analysis and address model errors.
Error analysis is the process ML teams use to analyze where model predictions disagree with ground truth labels. A disagreement between model predictions and ground truth labels can be due to a model error (poor model prediction) or a labeling mistake (ground truth is wrong). In this section, we detail 3 workflows to surface model errors—edge cases on which the model is struggling—using Labelbox.
Follow the steps and examples below to learn how to use filters, metrics, and the projector view to surface model errors.
Use filters in the gallery view
-
Inside a model run, go to the gallery view by clicking the gallery icon in the top right.
- Optionally, click on Splits and select the Validation or Test split. Some machine learning teams prefer doing error analysis on the validation or test splits only, rather than on all the training data.
-
Filter the data rows to keep only disagreements between model predictions and ground truth annotations. To do so, you can add a Metrics filter to keep only data rows with low metrics (IOU between 0 and 0.5 in the example below). In the image model example below, you can see that 307 data rows matching these filters are surfaced.
-
You then have a couple of options for the next step:
- Surface data rows where the disagreement is the highest. To do so, you can sort data rows by increasing metrics (IOU in the example). Predictions that have the lowest metrics are likely to be model errors.
- Surface data rows where the model is least confident. To do so, you can sort data rows by increasing order of confidence. This assumes you have uploaded model confidence as a scalar metric to the model run. Predictions that have low metrics (IOU) and low model confidence are likely to be edge cases on which the model is struggling.
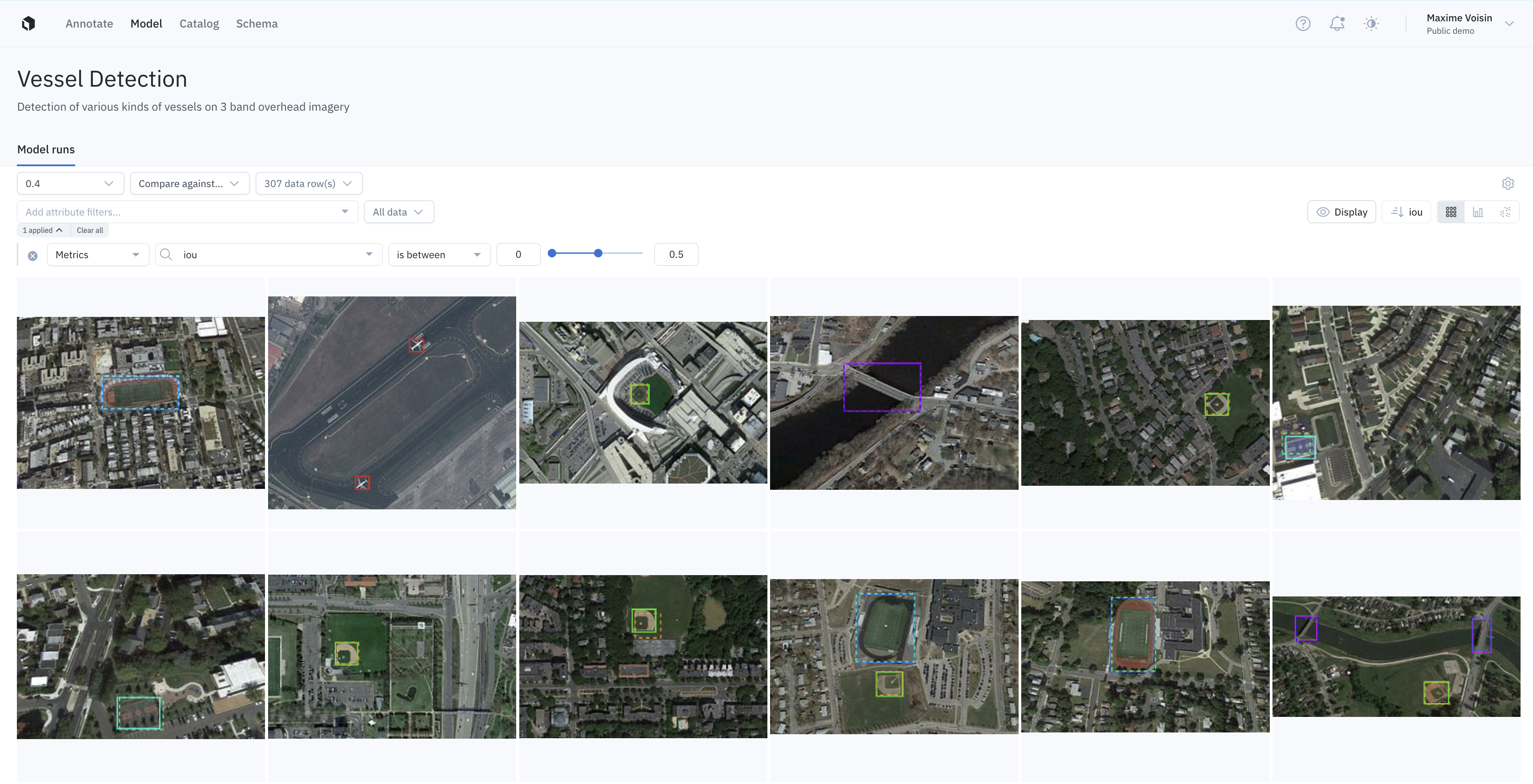
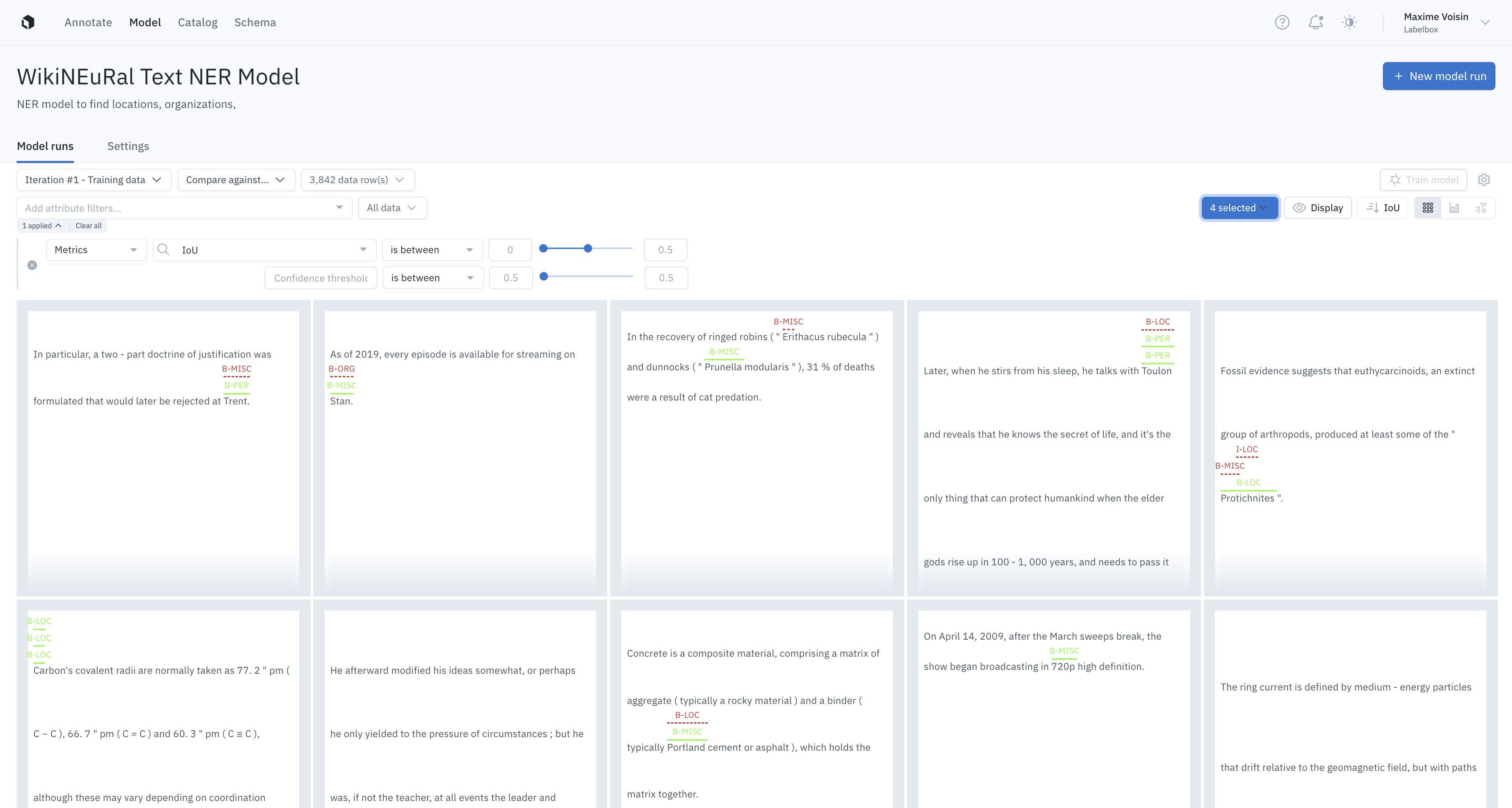
Filter and sort to keep the largest disagreements between model predictions and ground truth annotations.
- Then, manually inspect in detail some of these surfaced data rows. The goal is to find patterns of edge cases on which the model is struggling. It is common practice to manually inspect hundreds of data rows to find these patterns. To do so, click on a thumbnail to open the detailed view. For the best error analysis experience, change the display setting to Color by feature. This way, you can easily visualize where predictions and labels disagree.
In this image example, you can see several occurrences of data rows where the model predicts a basketball_court
instead of a ground_track_field
. You found a pattern of model failures that can be described as follows: "The model seems to struggle to distinguish ground track fields and basketball courts, especially when they have green and brown colors".
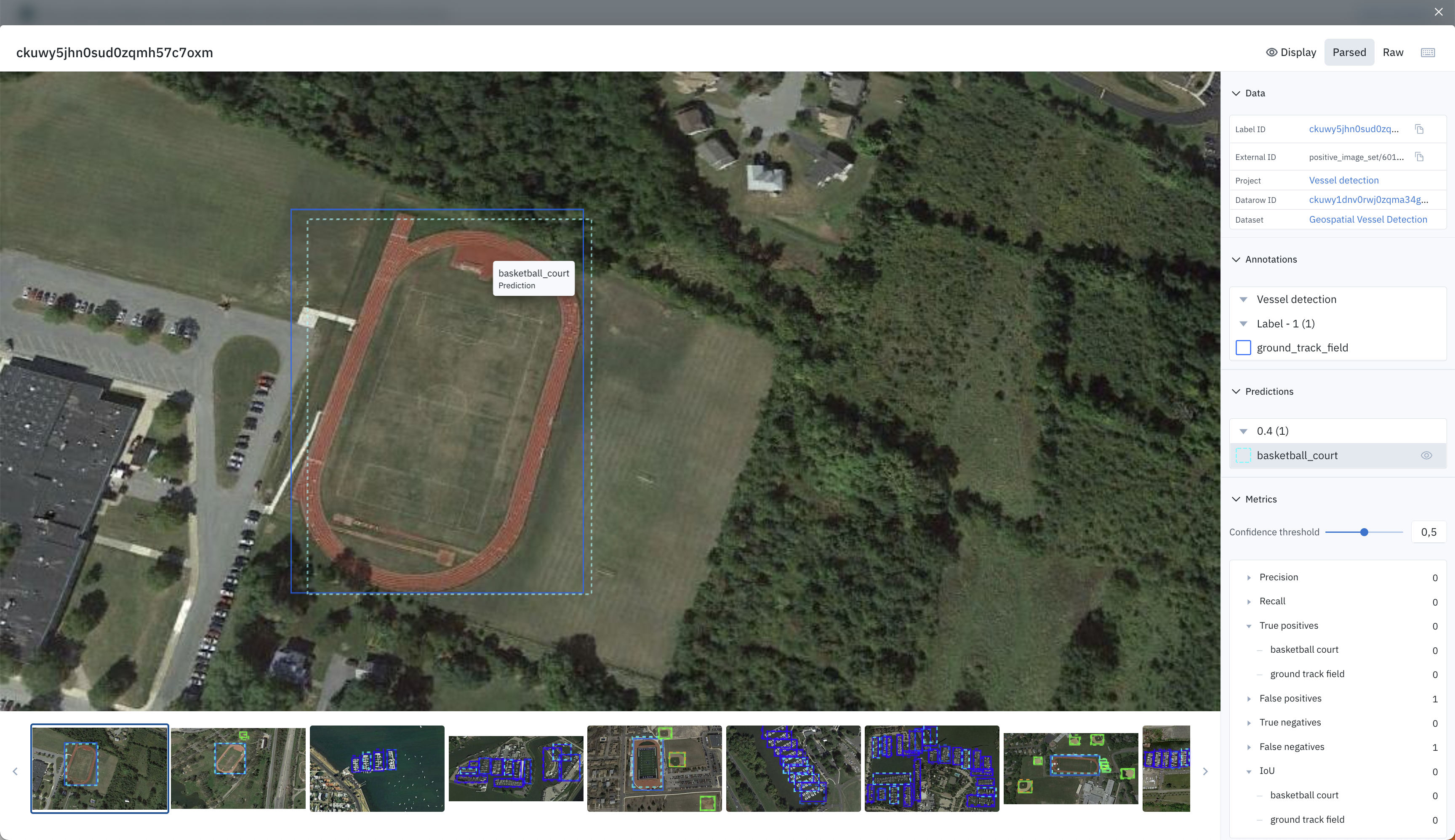
The detail view helps you inspect disagreements. The goal is to find patterns of model failures.
In this text example, you can see several occurrences of data rows where the model makes poor predictions on scientific words such as "Gelidium". You found a pattern of model failures (i.e., the model seems to struggle with text related to scientific concepts).
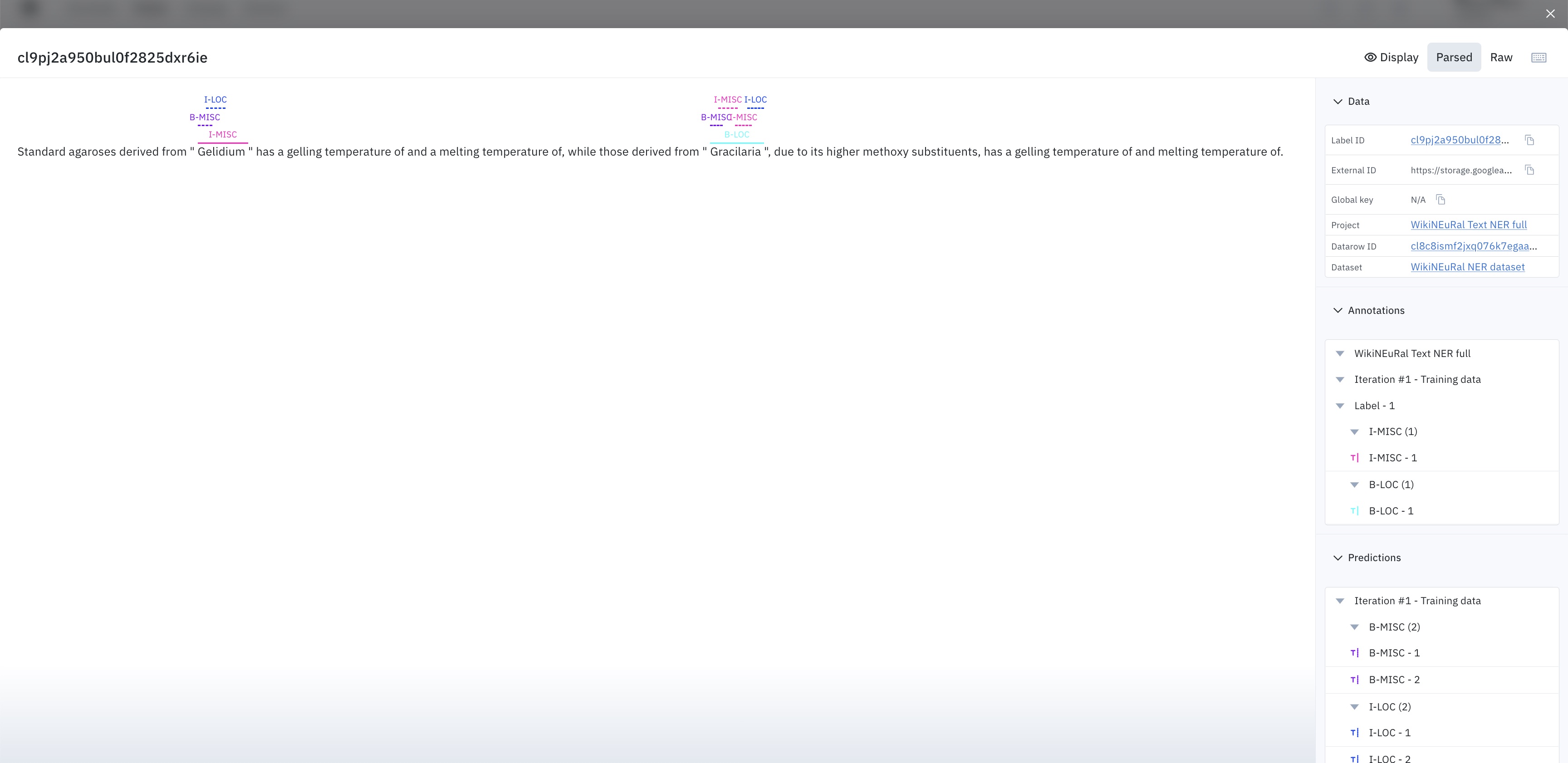
The detail view helps you inspect disagreements. The goal is to find patterns of model failures.
- Double-check that you have found a pattern of model failure. For example, in the image example, we filter to keep only data rows that contain a
basketball_court
annotation or aground_track_field
annotation and have low IOU. This surfaces many examples of the exact edge case we discovered above: "The model struggles to distinguish ground track fields and basketball courts, especially when they have green and brown colors".
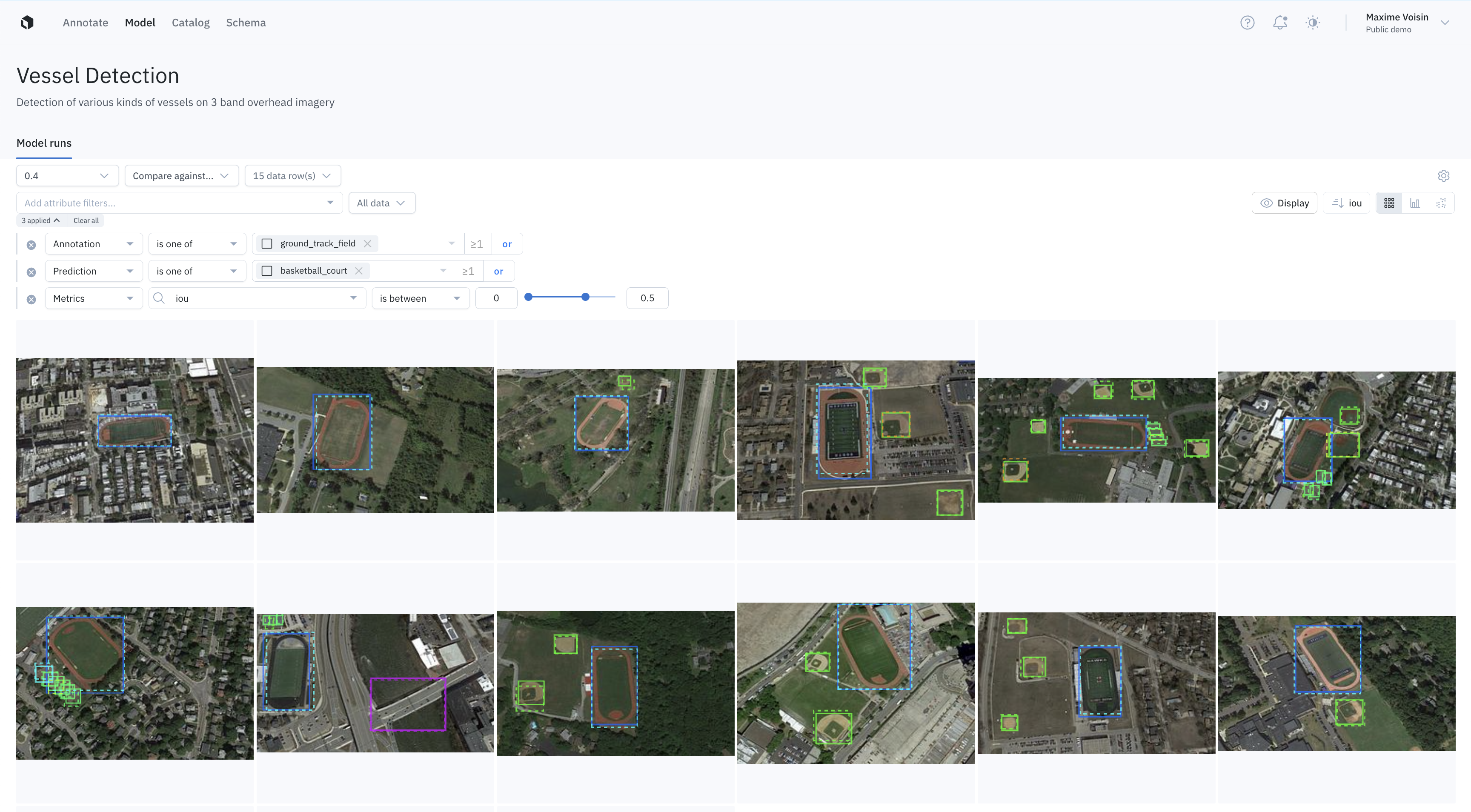
Many ground_track_field
s and basketball_court
s are being mispredicted (low IOU). This is a pattern of model failure.
By browsing through examples in this pattern of model failure, you can see that many basketball courts have brown and green colors, just like ground track fields.
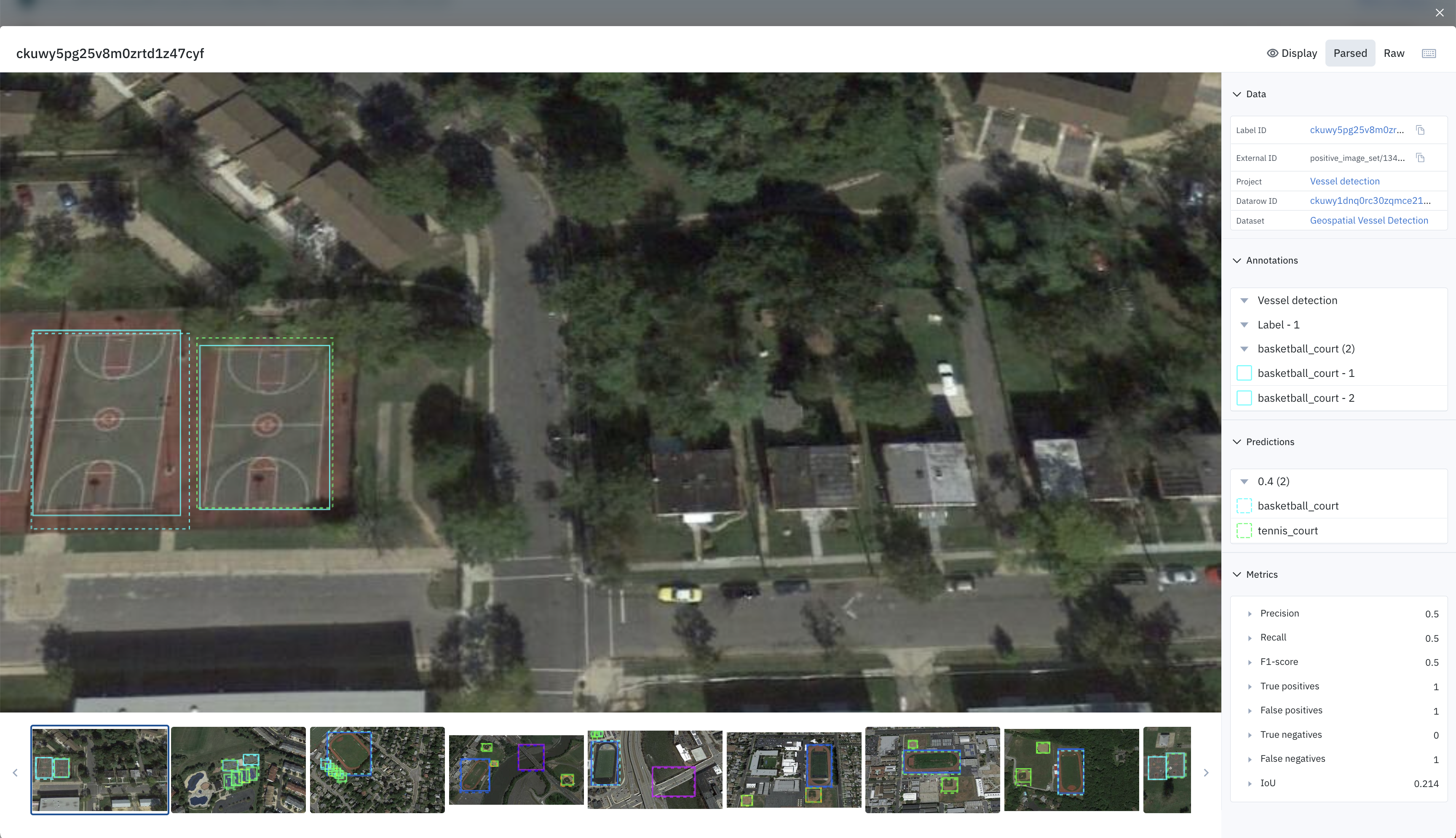
Labelbox helps you find patterns of model failures. In this case, the model struggles to distinguish ground track fields and basketball courts, especially when they have green and brown colors.
For example, in the text example, we filter to keep only data rows that contain a miscellaneous
annotation and that have low IOU. This surfaces many examples of the exact edge case we discovered above: "The model struggles to make accurate NER predictions on scientific concepts".
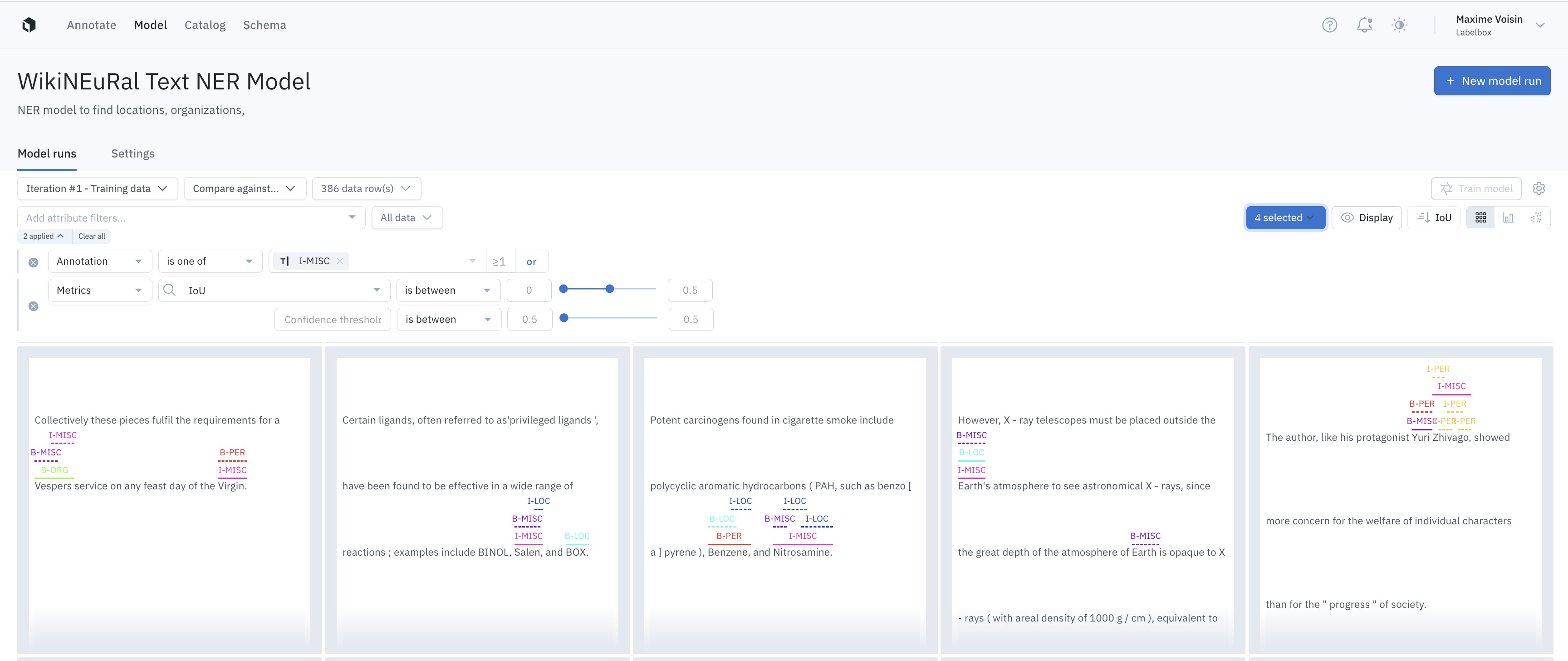
Labelbox helps you find patterns of model failures. In this case, struggles to make accurate NER predictions on scientific concepts.
After you surface edge cases on which the model is struggling and find a pattern of model failure, you can take action to fix model errors and improve model performance.
Use model metrics
The metrics view is a powerful tool for performing error analysis.
By looking at the scalar metrics, you may notice that the model is struggling to detect basketball_court
ground truth annotations. Then, you can click on the histogram bar corresponding to basketball courts and the gallery view will open. with filtering and sorting activated to show data rows in the basketball_court
bar of this histogram.
This is an alternative to steps 1-4 described above in the Use filters in the gallery view section.
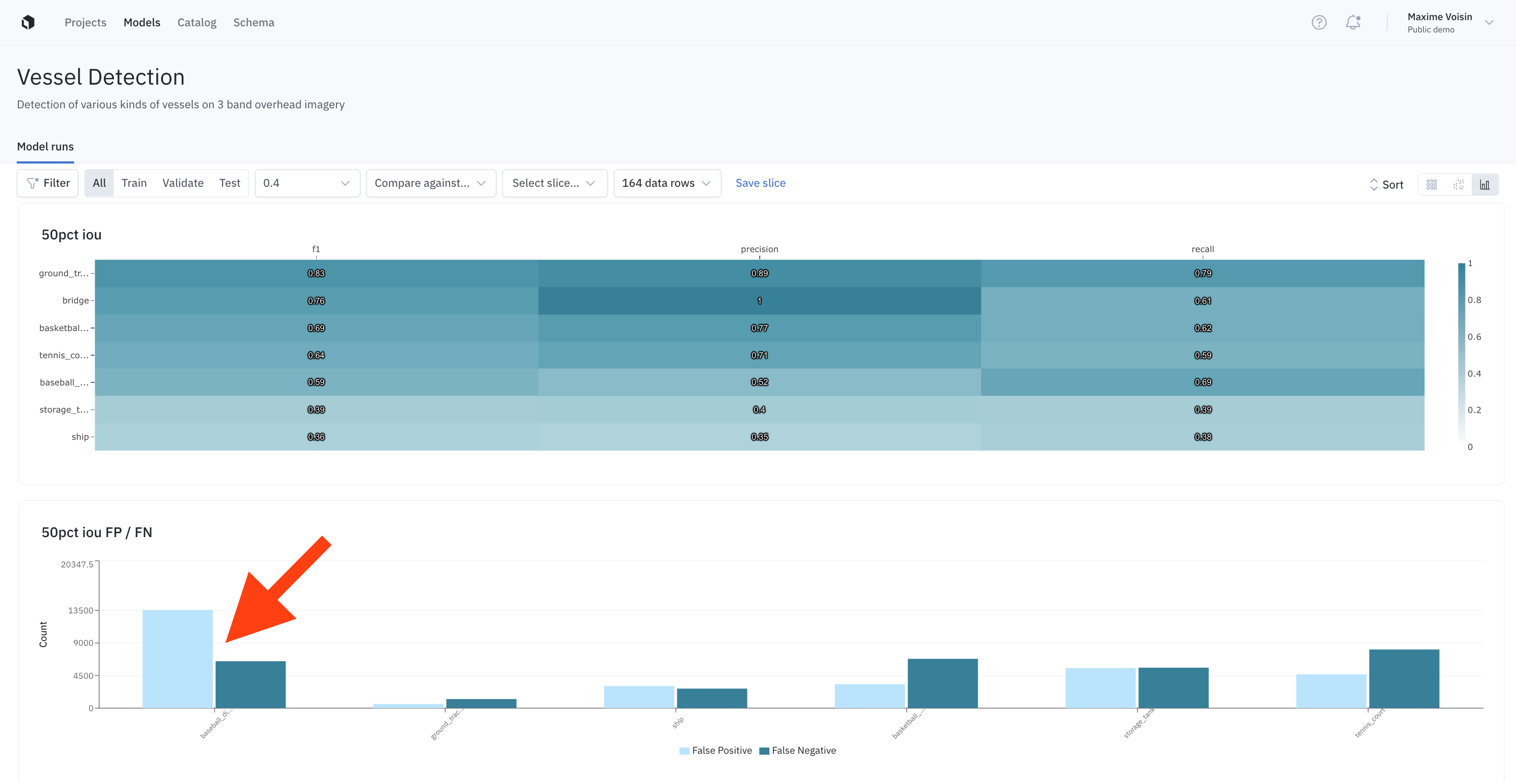
The metrics view is a good way to identify classes on which the model is struggling.
Use the projector view
The projector view is a powerful way to perform error analysis.
In the image below you can see data rows containing a ground_track_field
annotation and those containing a basketball_court
annotation overlap. The two classes are not easy to separate in the embedding space. This is an indicator that the model is likely to struggle with the data rows at the intersection of the two clusters.
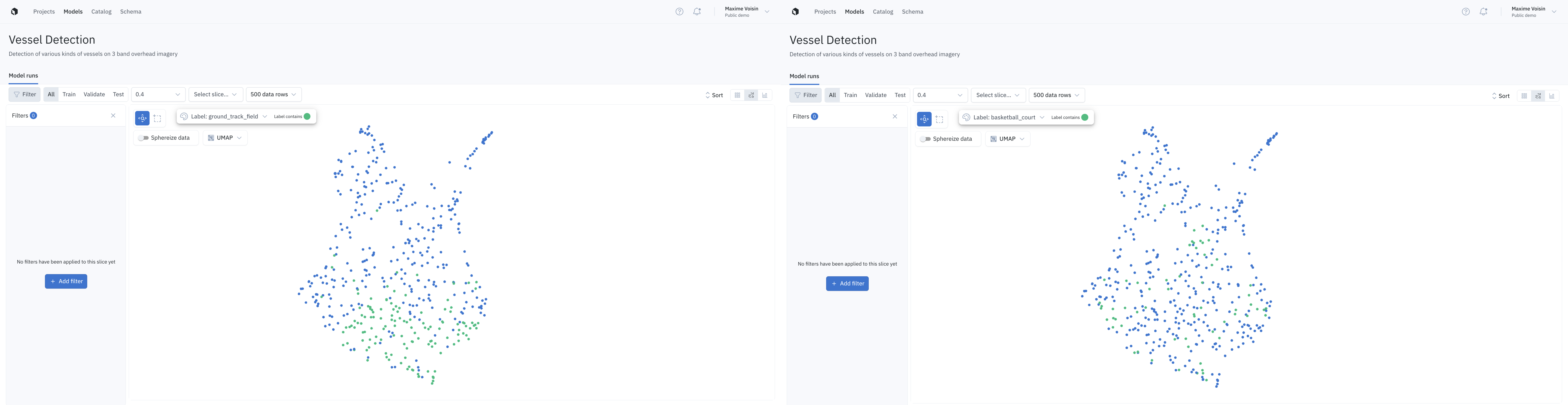
Use the projector view to identify intersections of clusters where the model may be struggling.
In the projector view, you can select the data rows that are at the intersection of the basketball_court
cluster and the ground_track_field
cluster. Once the data rows are selected, you can switch back to the grid view, and inspect these data rows.
This is an alternative approach to steps 1-4 described above in the Use filters in the gallery view section.
Fix model errors
Once you find a pattern of model errors, you can take action to improve your model. Here is an example of a data-centric iteration to improve model performance:
- Select data rows on which your model is struggling.
- Open the selected data rows in Catalog by clicking on [n] selected > View in Catalog. You will then be redirected to a filtered view of your Catalog showing only the previously selected data rows.
- Use similarity search to surface data similar to this pattern of model failures among all of the data in your Catalog. Optionally, you could create a slice that will automatically collect any similar data uploaded in the future.
- Next, you could filter on Annotation > is none to surface only unlabeled data rows. Labeling this high-impact data and then re-training your model is a powerful way to boost model performance.
- Create a batch and send it to a labeling project.
Once this data has been labeled in Labelbox, you can create a new model run, include these newly labeled data rows in your data splits, and retrain your machine learning model to improve its ability to detect these difficult cases.
Updated 11 months ago