Import ground truth
Learn how to import your ground truth data from internal or third-party tools into Labelbox.
Check out these end-to-end developer guides to learn how to import annotations.
Developer Guides:
- Import image annotations
- import video annotations
- Import text annotations
- Import geospatial annotations
- Import document annotations
- Import conversational text annotations
- Import audio annotations
- Import HTML annotations
Overview
This functionality allows you to bulk import your ground truth annotations from an external or third-party labeling system into Labelbox Annotate. Using the label import API to import external data is a useful way to consolidate and migrate all annotations into Labelbox as a single source of truth.
The steps for importing annotations as ground truth are very similar to the steps for importing annotations as pre-labels (see Model-assisted labeling (MAL)). However, importing annotations as ground truth is a bulk operation that is meant to be used when you are migrating to Labelbox from a third-party platform and is typically not part of your everyday workflow in Labelbox.
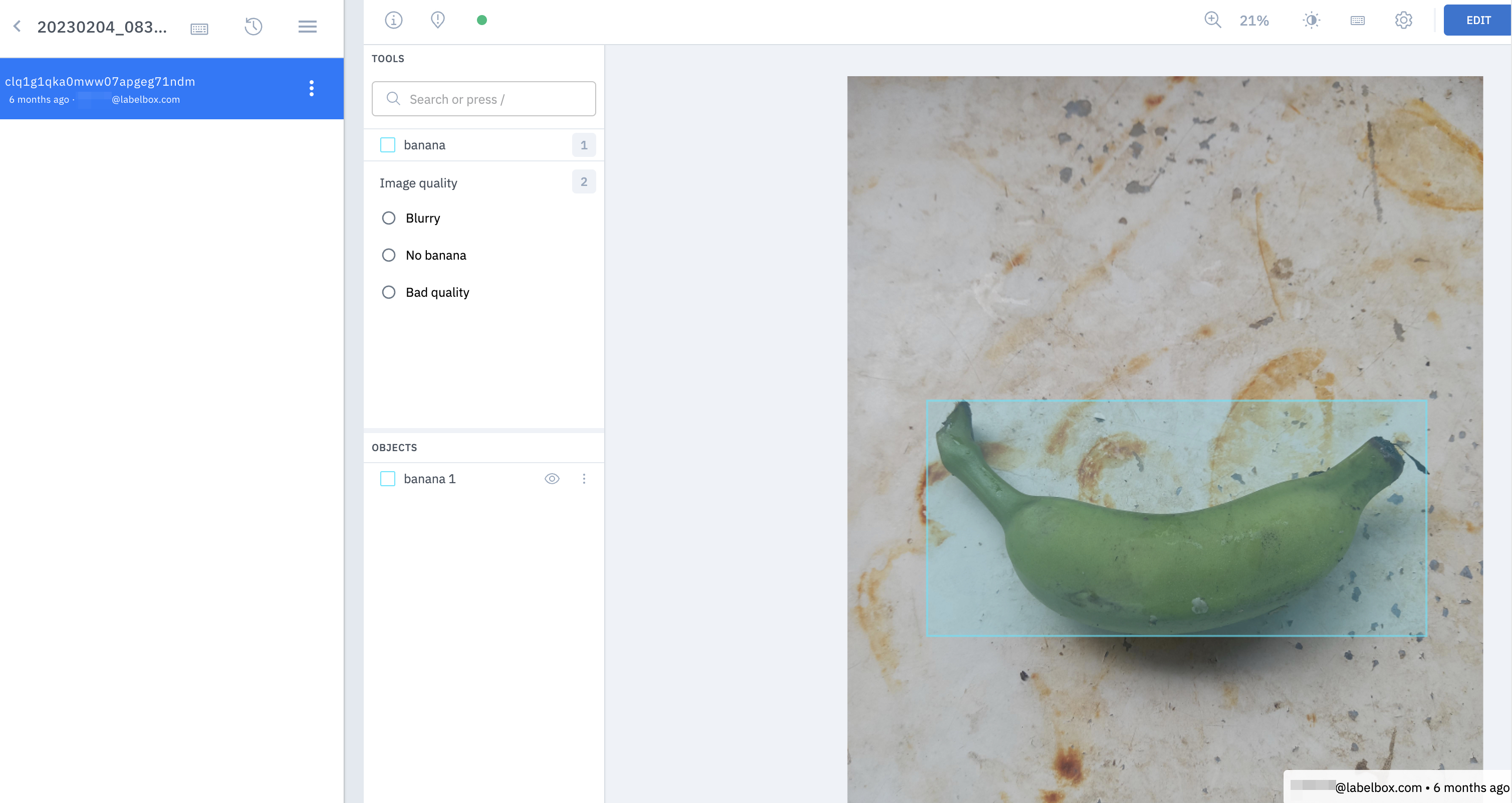
Data row browser view of imported ground truth annotations. Ground truth annotations will show as "Done" in your project
How to import annotations as ground truth
Generate Python SDK import annotation code
Based on your project's ontology, you can generate code snippets to import your annotations to the Labelbox platform. These code snippets serve as boilerplate code for your workflow and can help speed up the below steps. To find these snippets:
- Navigate to the Import labels tab of your project
- Select "Step 1: Create annotation payload" and "Step 2: Import annotations payload" using the dropdown.
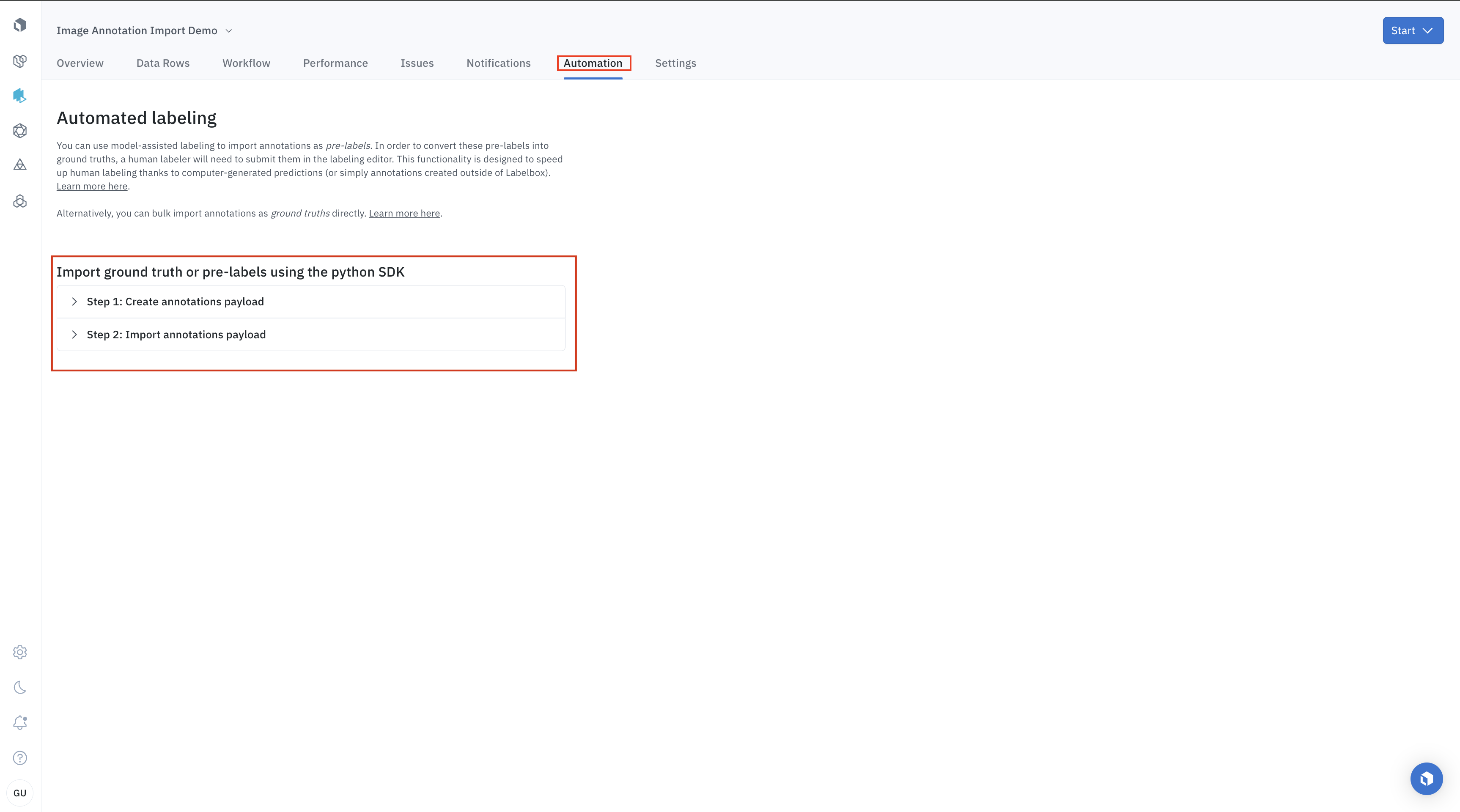
Step 1: Import data rows
To import annotations as pre-labels, you'll need to have a set of data rows to attach the annotations to. If you do not already have a set of data rows, you'll need to create data rows by importing a dataset to Catalog.
To learn how to import data rows via the Python SDK (Step 1), see this tutorial.
To learn more about creating data rows, see Create a dataset in Catalog.
Step 2: Create/select an ontology
When you import a set of annotations, you'll need to specify the ontology (also called taxonomy) that corresponds to the set of annotations. If the project ontology already exists in Labelbox, you may select the ontology that fits your annotations. If the ontology does not exist in Labelbox yet, you'll need to create an ontology.
To learn how to create an ontology via the Python SDK (Step 2), see this tutorial.
To learn more about ontologies, see Create/modify ontologies.
Step 3: Create a labeling project
Before you can import your annotations, you'll need to make sure you have a project to connect these annotations to. You cannot simply import annotations without specifying which project they'll be associated with. Oftentimes, you will already have a project set up with the correct ontology for your set of annotations. However, If you do not already have a project, you will need to create a project and attach the ontology that fits your annotations.
To learn how to set up a labeling project via the Python SDK (step 3), see this tutorial.
To learn more about creating projects, see Create a project.
Step 4: Send a batch of data rows to the project
Now that you have your project and ontology configured, you'll need to send a subset of data rows (i.e., a batch) to the project's labeling queue. This is the batch of data rows you will be attaching the annotations to.
To learn how to create a batch via the Python SDK (Step 4), see this tutorial.
To learn more about batch mode, see our docs on Batches.
Step 5: Create the annotations payload
After you have successfully configured your project with the correct ontology and selected a batch of data rows to attach the annotations to, you are ready to prepare the annotation payload. To do this, you will need to use our Python SDK. Each imported annotation will need to reference an annotation class within the ontology (see step 2 above) and a specific Data Row ID. Labelbox supports two formats for the annotations payload: NDJSON and Python annotation types.
Use the table below to find an annotation payload sample for your asset type. The "-" symbol indicates that importing as prelabels is not supported for that annotation/asset type combination. To learn how to create an annotation payload via the Python SDK, see this tutorial.
Image | Video | Text | Documents | Geospatial | Audio | Conversational text | |
---|---|---|---|---|---|---|---|
Bounding box | Payload | Payload | N/A | Payload | Payload | N/A | N/A |
Polygon | Payload | - | N/A | N/A | Payload | N/A | N/A |
Point | Payload | Payload | N/A | N/A | Payload | N/A | N/A |
Polyline | Payload | Payload | N/A | N/A | Payload | N/A | N/A |
Segmentation mask | Payload | Payload | N/A | N/A | - | N/A | N/A |
Text entity | N/A | N/A | Payload | Payload | N/A | N/A | Payload |
Classification - Radio | Payload | Payload | Payload | Payload | Payload | Payload | Payload |
Classification - Checklist | Payload | Payload | Payload | Payload | Payload | Payload | Payload |
Classification - Free-form text | Payload | - | Payload | Payload | Payload | Payload | Payload |
Relationship | - | - | - | - | - | N/A | - |
Step 6: Import the annotation payload
After you create your ground truth annotations payload, the final step is to submit the import job. Use this Python example to learn how to do this.
To learn how to import the annotations payload (Step 6) via the SDK, see this tutorial.
Best practices
- Before you begin a new import job to import annotations to a data row, make sure there are no existing MAL annotations on the data row. Duplicate import jobs may overwrite existing labels or result in unexpected behavior.
- When you run an import job, the activity page in Labelbox will not reflect any changes until the entire job is complete.
Billing
You can view the number of annotations imported for billing purposes by visiting the billing usage page. Please note that the billing system may indicate different counts for certain annotation types when compared to the annotation count on the project overview page. This is expected behavior.
To view your annotation usage, click on your initials in the bottom left corner. Then, select Workspace settings.
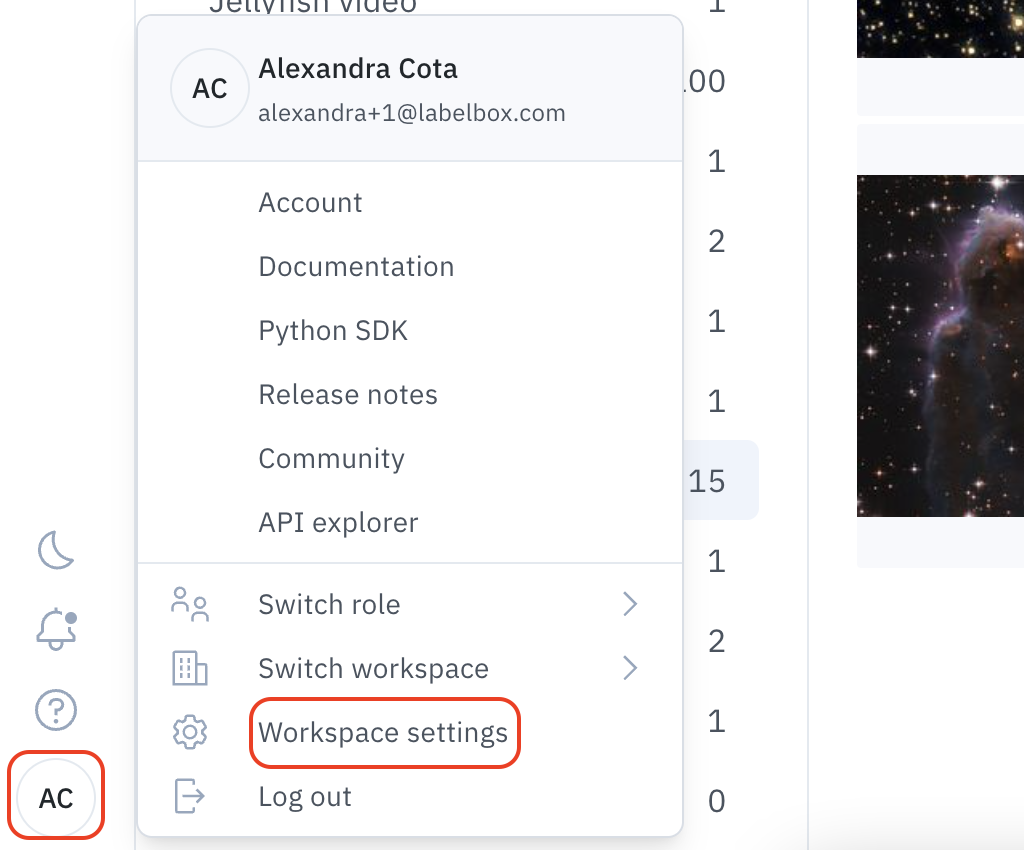
From there, navigate to the Usage tab.
Updated 2 months ago