Overview
Train, test, and evaluate your models with the Model product.
The Model product is designed to help you achieve the following:
- Easily prepare and version training data
- Launch model training experiments
- Diagnose the performance of your machine learning models and rapidly improve their performance
A Model can be used in conjunction with our Catalog and Annotate products.
How Model works
Labelbox recognizes that machine-learning teams need a model experiment management system in order to efficiently collaborate with their team members and make their experiments reproducible. You can think of the Model product as your command center for tracking all datasets and configurations at each data-centric iteration.
The Model product also provides a framework for comparing your models across multiple experiments. A model run is a representation of a model training experiment that contains a data snapshot (data rows, annotations, and data splits) at each iteration. By comparing model runs, you can visualize which configurations work best for your model and identify the model with the best performance. You can also use this framework to make sure you are making progress in your experiments.
What is versioned?
When you create a model run, Labelbox will automatically create a snapshot of data (including data rows, labels, and data splits) that cannot be changed after you upload model predictions. Even if the annotations or data rows are updated later on, the original snapshot version of the data will be preserved.
After you upload model predictions, given you have ground truth, Labelbox will automatically compute and version the model metrics for you.
Type | Versioned |
---|---|
Data | Data rows, labels (ground truth), and data split |
Model | Model metrics and model configs |
How can I retrieve the data snapshot?
Follow the steps in Export model run data.
Key terms
Key terms
Term | Definition |
---|---|
Model | A model is a large language model integrated by Foundry or your custom configuration specified by an ontology of data. |
Experiment | An experiment is a directory where you can create, manage, and compare a set of model runs related to the same machine-learning task. See Experiements . |
Model run | A model run is a model training experiment within a model directory. Each model run has its data snapshot (data rows, annotations, and data splits) versioned. You can upload predictions to a model run, and compare its performance against other model runs in the model directory. See Create a model run. |
Data split | You can split the selected data rows into train, validation, and test splits to prepare for model training and evaluation. See Splits. |
Data versioning | Each model run keeps its own versioned data snapshot. The snapshot contains the data rows, annotations, and data splits. It is immutable, meaning it remains the same even if new annotations are added or existing annotations are updated. You can export it from the model run to train or use it to reproduce a model. |
Model config (hyperparameters) | Each model run will keep a version of its model configurations (such as hyperparameters), and model type. See Model runs. |
Model training | Export the model run snapshot from Labelbox and train a model in your custom ML environment. See Export model run data (beta). |
Error analysis | Error analysis is the process through which ML teams analyze where model predictions disagree with ground truth labels. A disagreement can be a model error (poor model prediction) or a labeling mistake (ground truth is wrong). See Improve model performance. |
Slice | A slice represents a subset of your training data bound by a common characteristic. From the Model tab, you can create slices to visually inspect your training data and view model metrics reported on each slice. See Slices. |
Active learning | Active learning is the process through which ML teams identify, among all their unlabeled data, which high-value data rows they will label in priority. Labeling these data rows will optimally improve model performance. See Prioritize high value data to label (active learning). |
Inspect annotations and predictions
Labelbox designed the Model product to be highly visual. You can use the gallery view to visually inspect and compare annotations and model predictions.
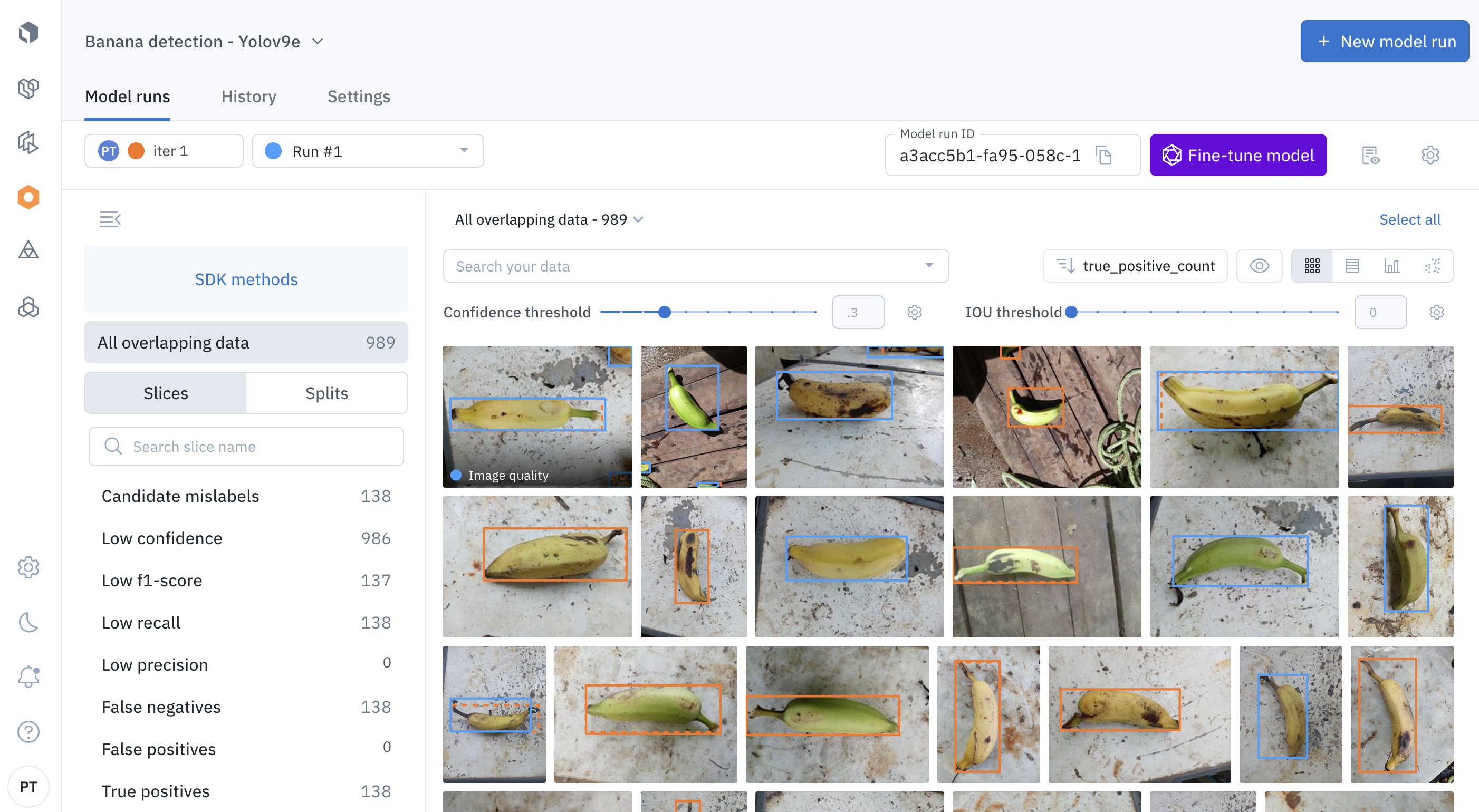
Compare, model prediction, ground truth across model version
Analyze performance
The Model product also provides a natively supported metrics view that enables you to evaluate the performance of your machine learning models. Model error analysis is the process by which you analyze where your model predictions disagree with ground truth labels. These quantitative metrics help you find low-performing slices of data so you can best understand how to tackle the root cause of the issue.
Understand the distribution
The Model product gives you several ways to diagnose and improve the performance of your models. Using the embeddings projector, you can visually inspect the data points to understand the distribution of your training/inference data, splits, annotations, and predictions.
With each data-centric iteration, you can improve your model performance by identifying model challenge cases, prioritizing the right labels to fix them, finding & fixing labeling mistakes, and pre-labeling your data.
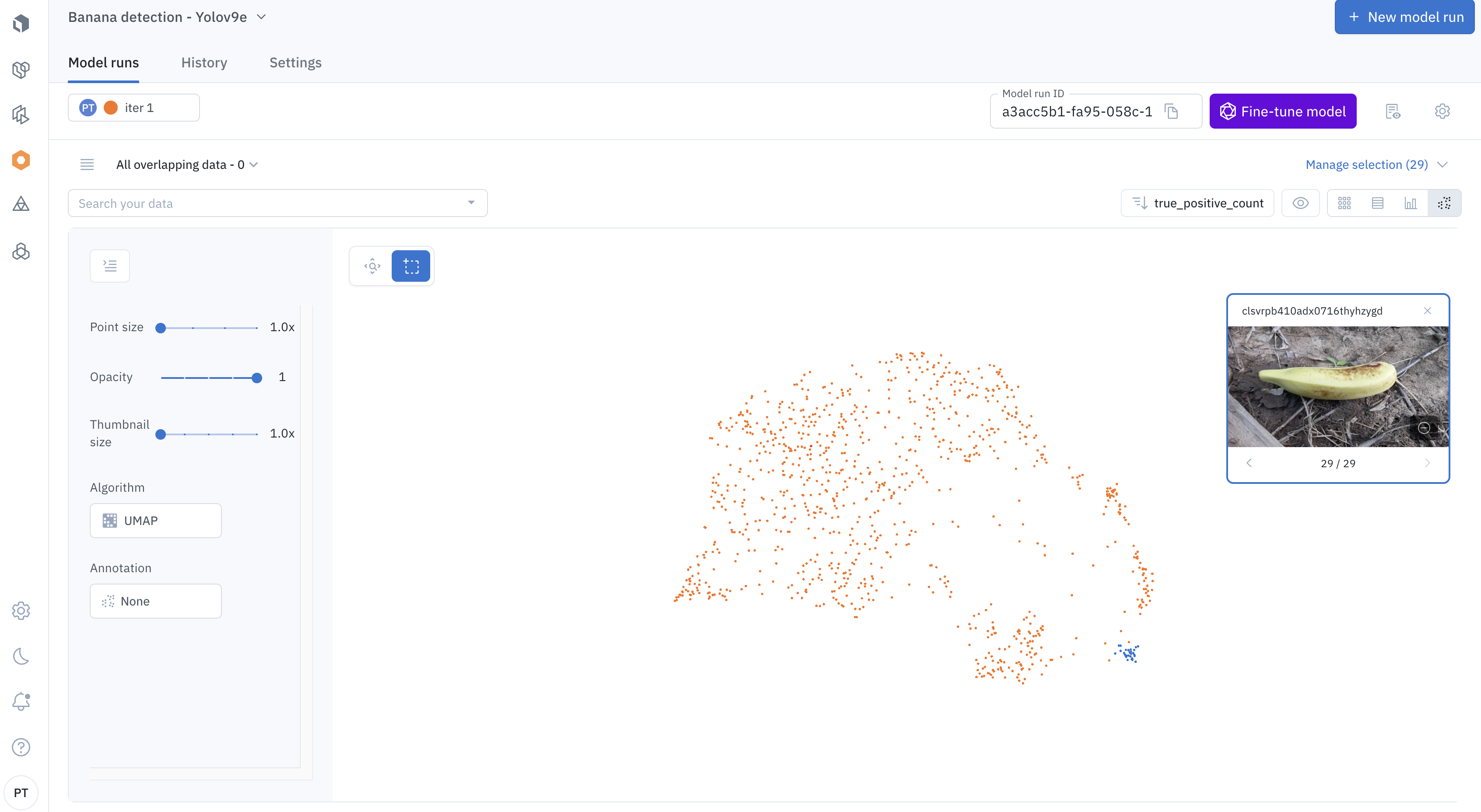
Understand the distribution of your training and inference data, splits, annotations, and predictions
Updated 7 months ago