Similarity search
Explore data with custom or off-the-shelf embeddings.
Training datasets that are carefully visualized, curated, and debugged are the most impactful for increasing model performance. However, these practices can be challenging to do on unstructured data (e.g., images, text, videos, PDF, etc.) because unstructured data is not queryable with something like SQL.
Labelbox's similarity search tool is designed to help you programmatically identify similar or dissimilar data. You can use this tool to mine data and look for examples of rare assets or edge cases that will dramatically improve your model performance. This similarity search engine gives your team an advantage by helping you surface high-impact data rows in an ocean of data.
The alternative to using Labelbox's similarity search engine is to build your own similarity search tool. However, building an in-house similarity search tool that scales to hundreds of millions of data points — and that provides results instantaneously in just one click — is difficult for even the most advanced machine learning teams.
We recommend that you use the native similarity search engine within our Catalog product.
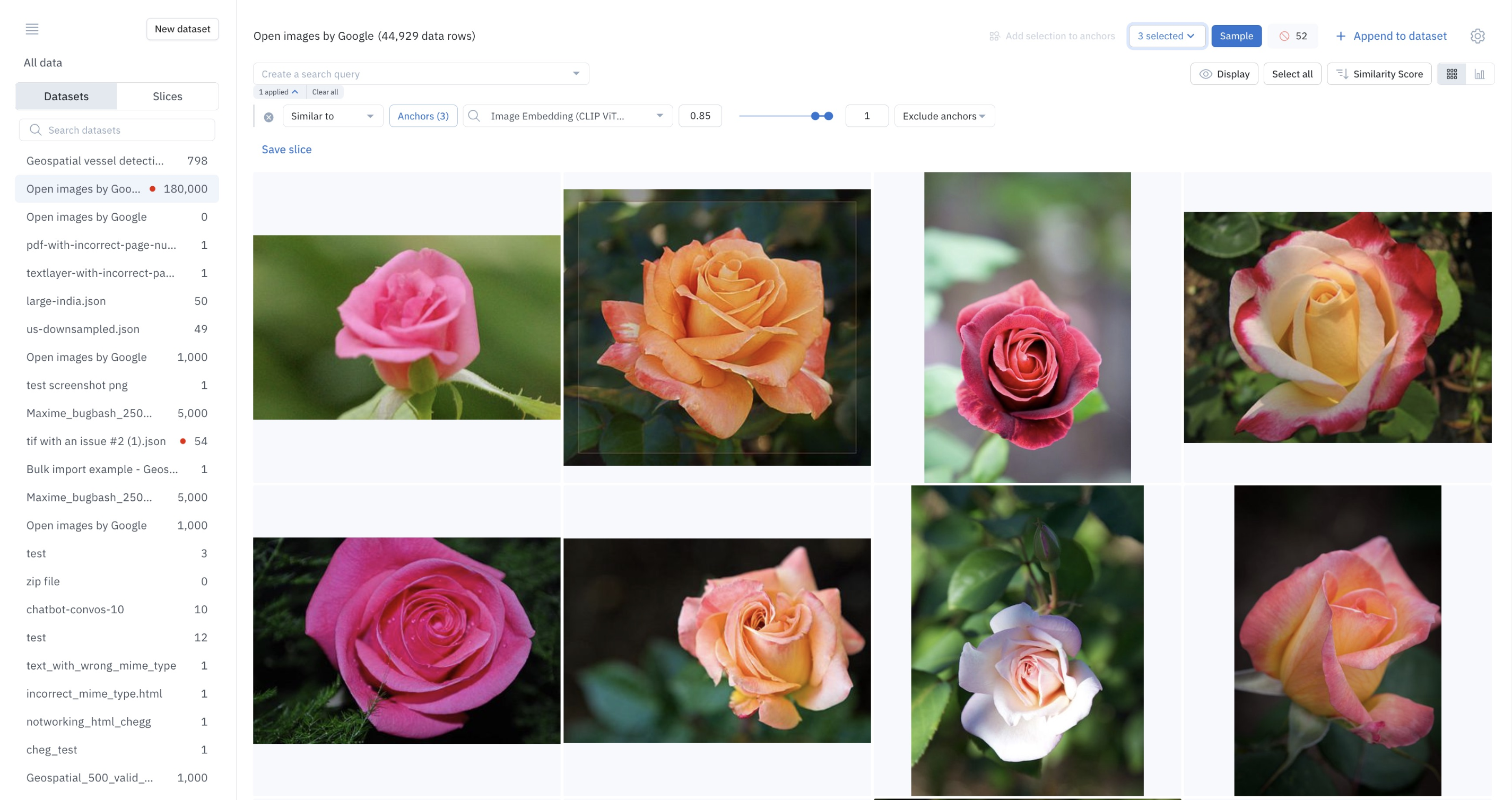
Use similarity search to programmatically surface similar data.
How similarity search works
Similarity search is powered by vector embeddings. A vector embedding is a numerical representation of a piece of data (e.g., an image, text, document, or video) that translates high-dimensional data into a lower-dimensional space.
There are many ways to create vector embeddings for data. However, generating embeddings via neural networks is the most common and effective approach. Most neural networks are designed to produce structured outputs like bounding boxes or classifications.
However, in order to produce the final prediction, a model will undergo a series of internal states before the final output is produced. Embeddings work by extracting the internal state and using it as a representation of the data row. In other words, the neural network acts as a feature extractor: it extracts an embedding vector that contains rich information about the data row.
The data rows that are used as input in a similarity search are called anchors. When you search for similar data rows, Labelbox surfaces and returns the data rows whose embeddings are closest to those of anchor data rows. Labelbox uses Manhattan distance (also known as L1 distance) to measure similarity.
Supported embeddings
Labelbox automatically computes off-the-shelf embeddings for the data types noted below. To do so, Labelbox uses neural networks trained on publicly available data. Off-the-shelf embeddings provide a useful starting point to explore your data and perform similarity searches.
Asset type | Off-the-shelf | Custom |
---|---|---|
Image | CLIP-ViT-B-32 (512 dimensions) | Up to 2048 dimensions per embedding; up to 100 custom embeddings per workspace. |
Video | Google Gemini Pro Vision . First two (2) minutes of content is embedded. Audio signal is not used currently. This is a paid add-on feature available upon request. | Up to 2048 dimensions per embedding; up to 100 custom embeddings per workspace. |
Text | all-mpnet-base-v2 (768 dimensions) | Up to 2048 dimensions per embedding; up to 100 custom embeddings per workspace. |
HTML | all-mpnet-base-v2 (768 dimensions) | Up to 2048 dimensions per embedding; up to 100 custom embeddings per workspace. |
Document | CLIP-ViT-B-32 (512 dimensions) and all-mpnet-base-v2 (768 dimensions) | Up to 2048 dimensions per embedding; up to 100 custom embeddings per workspace. |
Tiled imagery | CLIP-ViT-B-32 (512 dimensions) | Up to 2048 dimensions per embedding; up to 100 custom embeddings per workspace. |
Audio | Audio is transcribed to text. all-mpnet-base-v2 (768 dimensions) | Up to 2048 dimensions per embedding; up to 100 custom embeddings per workspace. |
Conversational | all-mpnet-base-v2 (768 dimensions) | Up to 2048 dimensions per embedding; up to 100 custom embeddings per workspace. |
View available embeddings on a data row
To find an exhaustive list of embeddings for a data row, go to Catalog and open the detailed view of the data row. In the detailed view under Metadata, you will find all embeddings available on the data row.
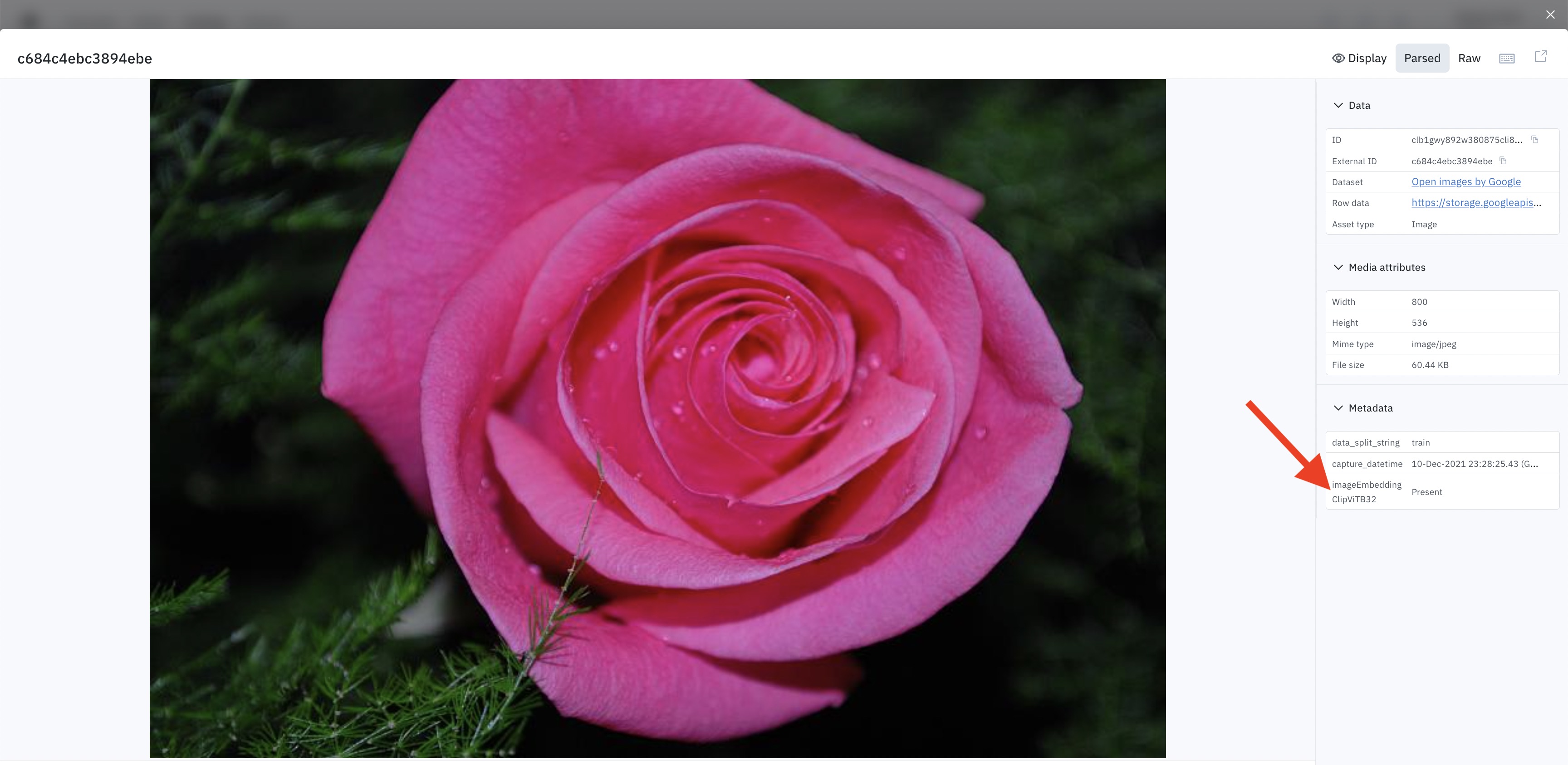
The detailed view of Catalog shows the list of embeddings available on a data row.
Upload custom embeddings
For instructions on how to upload custom embeddings using the Python SDK, please see our technical documentation here.
Search for similar data rows
Data rows that share common characteristics are represented by vectors that are close to each other in the embedding space. There are several ways to do a similarity search within the Catalog product.
Select the initial anchor
In the gallery view of Catalog, you can find similar data rows in just one click. To do this, hover over a thumbnail and an icon will show up in the bottom-right corner of the thumbnail. Click on it to find similar data rows.
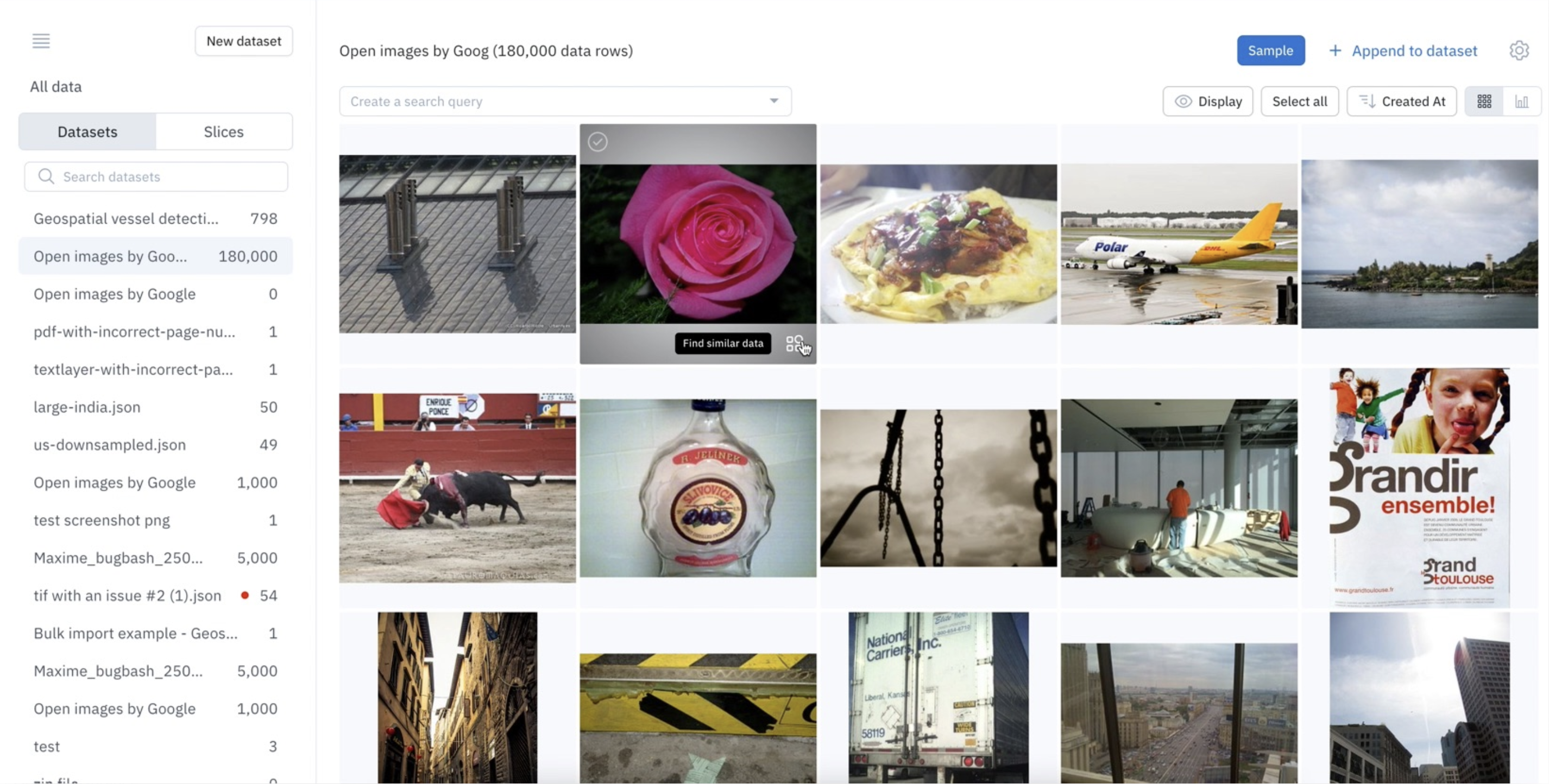
Launch a similarity search by clicking on the thumbnail.
Once you've clicked on the similarity search icon, Labelbox will automatically populate the Similar to filter and display data rows that are similar to the anchor data row in the gallery view.
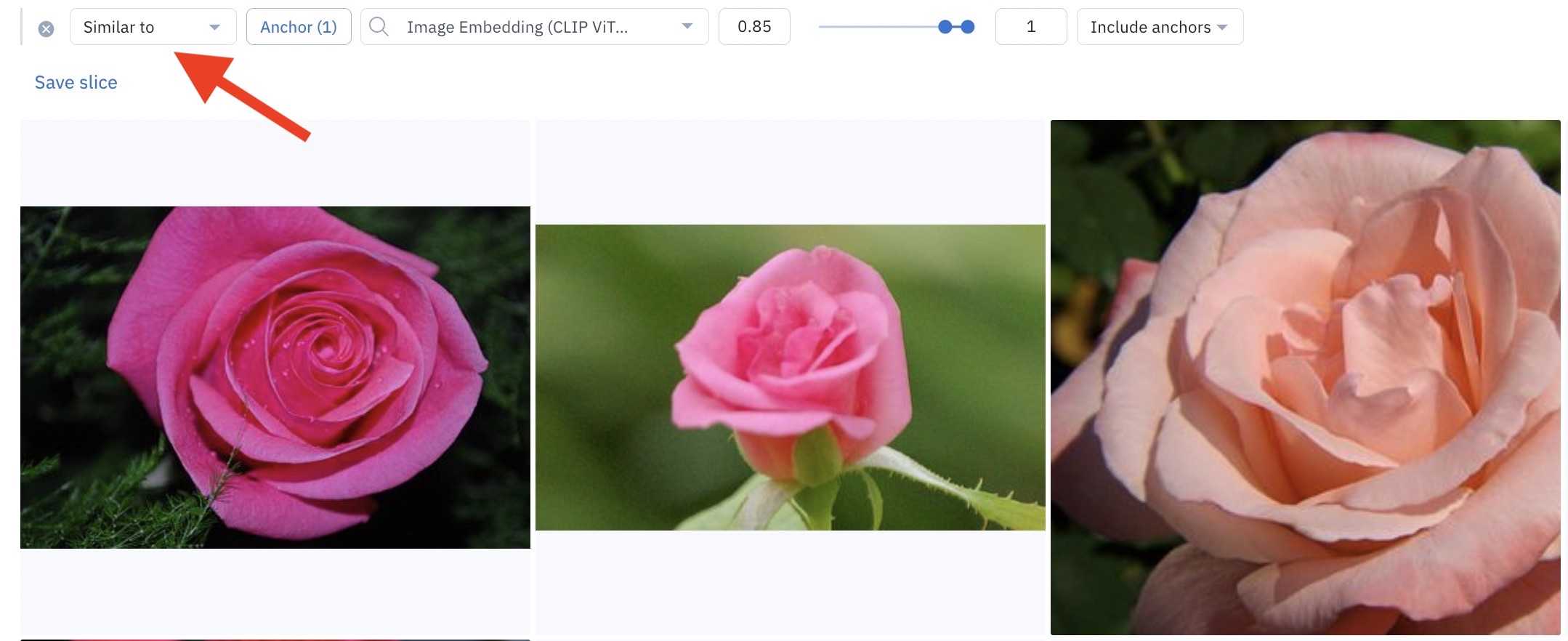
A similarity search shows up as a filter in Catalog.
Select multiple initial anchors
Alternatively, you can select multiple data rows as anchor data rows. To do this, go to the gallery view of Catalog, select multiple data rows, then click Similar to selection. Labelbox will then populate the gallery with similar data rows.
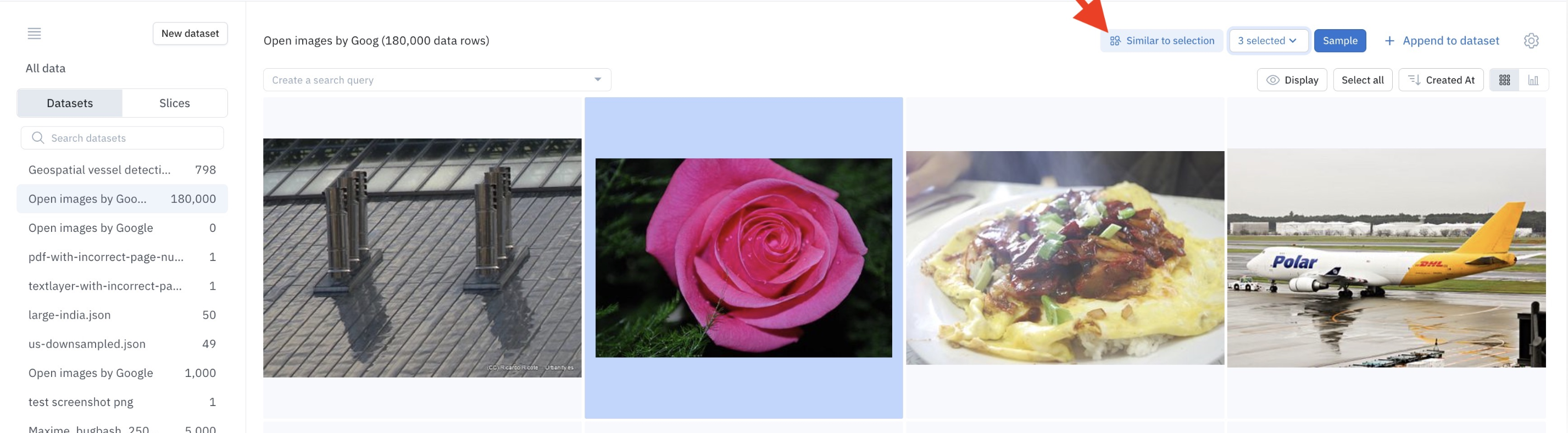
Launch a similarity search using multiple anchors
After you click Similar to search, Labelbox will automatically populate the Similar to filter and display data rows that are similar to the anchor data row in the gallery view.
Refine the similarity search
There are several approaches and tools available to powerfully refine your initial similarity searches.
Add anchors
While browsing through the results of a similarity search, Labelbox recommends refining the similarity search by adding more anchors. To do so, select the data rows of interest and click Add selection to anchors.
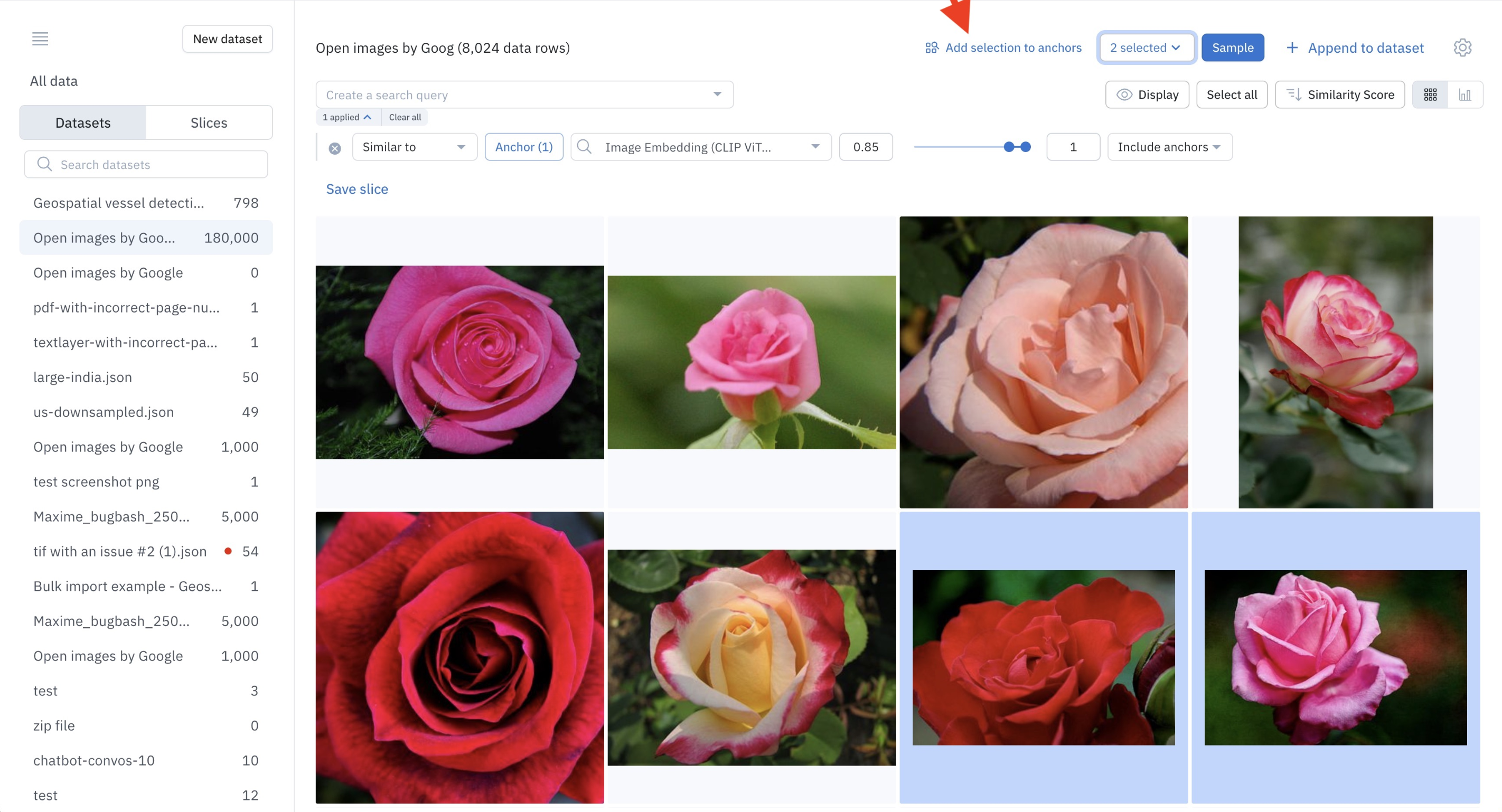
Add selected images as anchors to refine your similarity search.
Anchor limit
To view the max anchors allowed per similarity search, visit our Limits page.
Visualize anchors
Once a similarity search filter has been populated, you can visualize the anchors associated with it by clicking on Anchors (n).
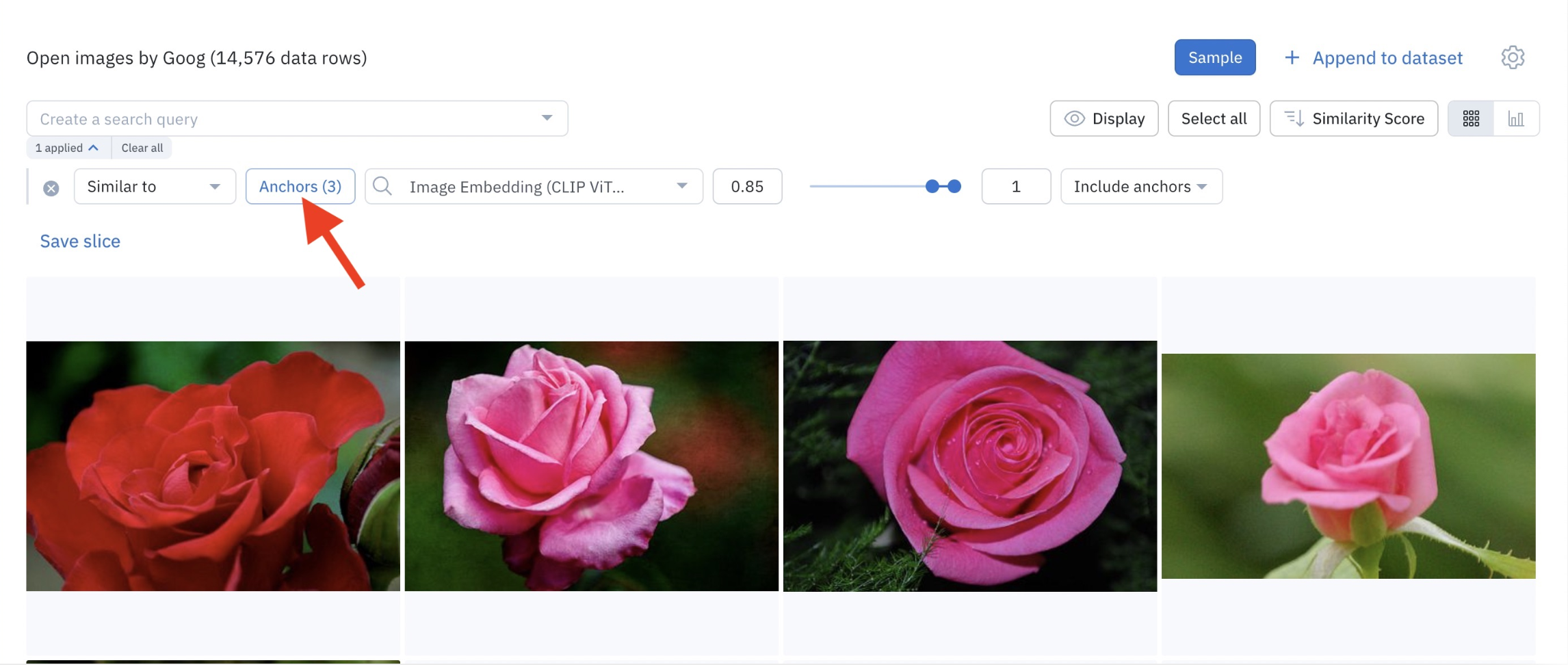
Click Anchors (n) to see the anchors.
Remove anchors
To remove one or more anchors from a similarity search, first visualize all anchors (see the previous paragraph), then hover on the thumbnail of the anchor to remove and click on the (—) icon.
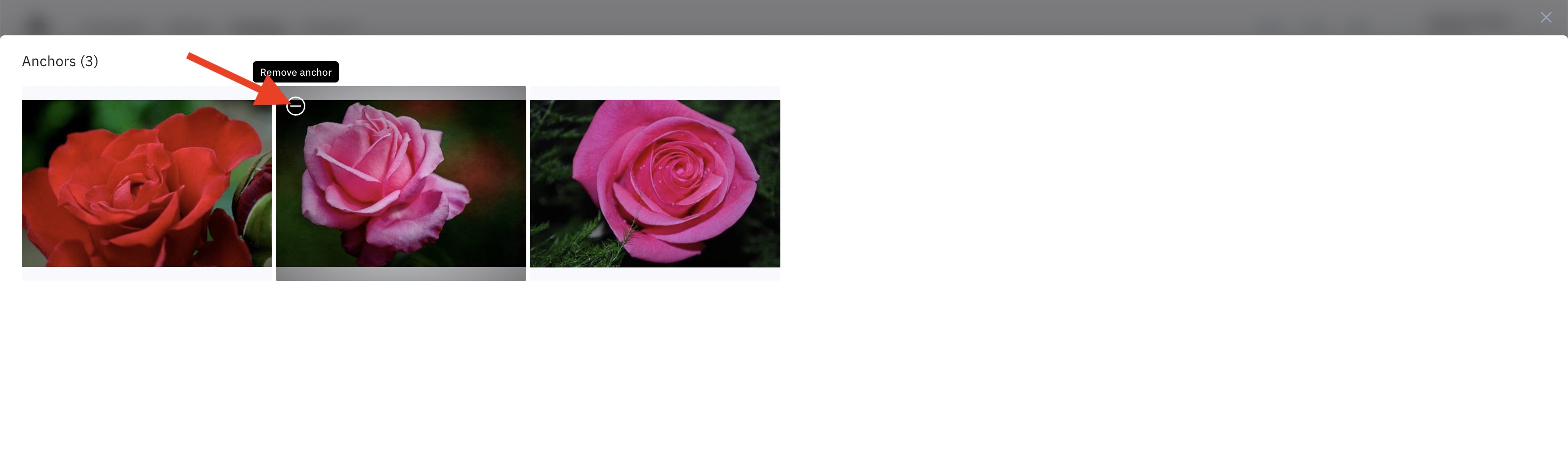
Remove one or more anchors from a similarity search.
Customize the range of similarity scores
Similarity search surfaces the data rows whose embeddings are closest to those of anchor data rows. This is measured using Manhattan distance (also known as L1 distance). It is a number between 0 and 1. The more similar the embeddings, the higher the similarity score.
By default, Labelbox returns embeddings with a similarity score between 0.85 and 1. You can customize this range by setting the minimum and maximum values of the similarity search slider.

Customize the results of the similarity search by specifying the range of similarity scores.
Specify an embedding
Similarity search relies on a choice of embedding. You can decide which embedding to use for similarity search. To do this, select an embedding from the dropdown in the Similar to filter.

Specify the embedding that powers the similarity search.
All off-the-shelf embeddings and custom embeddings can be used to power the similarity search.
Combine similarity search with other filters
You can combine similarity search with other filters in Catalog. Some filters are best used for targeting unstructured data and others are best for targeting structured data.
Combine natural language search with the following filters to target data rows by structured data:
Combine natural language search with the following filters to search unstructured data:
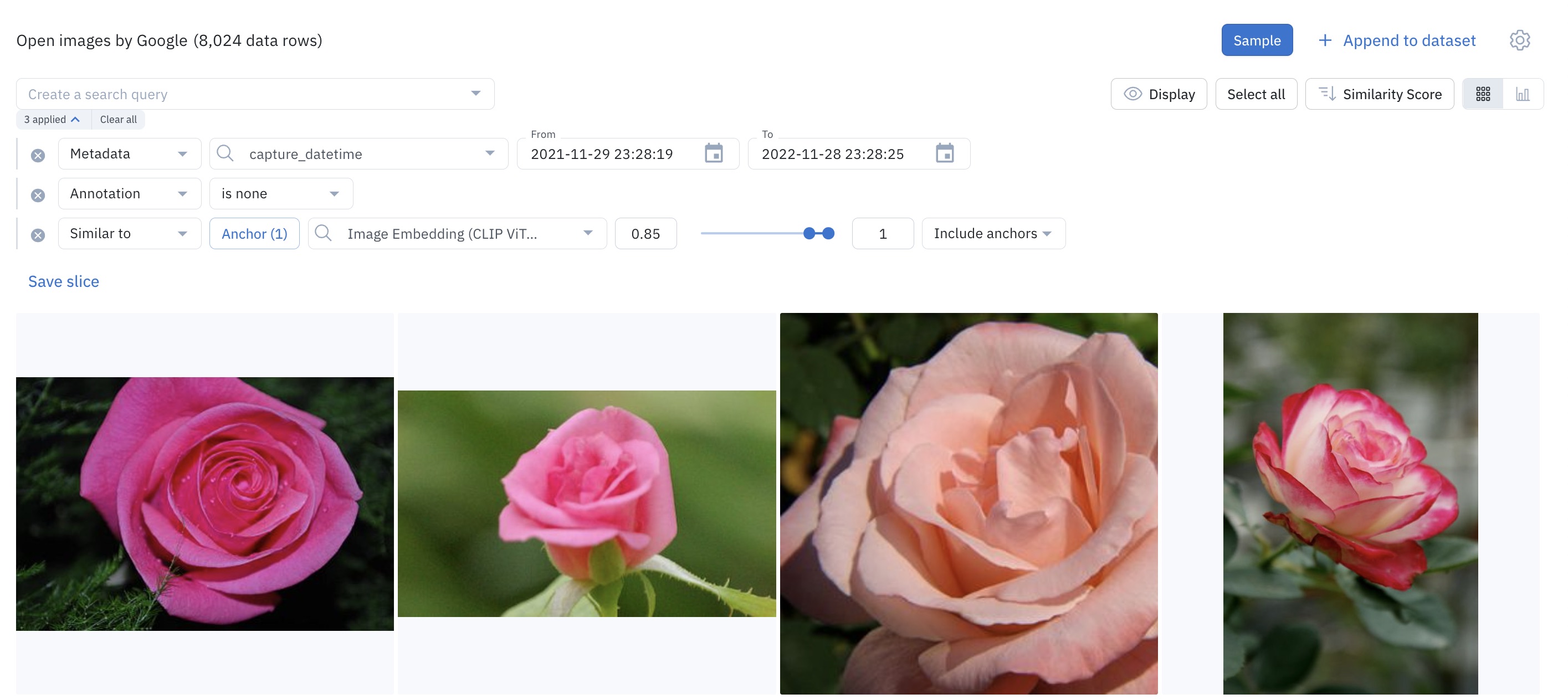
Similarity search can be used in conjunction with other filters to surface high-impact data.
Automate data curation with slices
After populating filters in Catalog, you can save these filters as a slice of data. When you save a filter as a slice, you will not need to populate the same filters over and over again. Slices are dynamic, thus any incoming data rows in Catalog will show up in the relevant slices.
Read through the following resources to learn how to take action on the filtered data:
Updated 6 months ago