Filters
A guide for filtering and sorting data rows in Catalog.
You can use the filters in Catalog to help you gather insights about your data, surface high-impact subsets of data rows, and remove unwanted data.
Supported attributes
Below are the attributes you can use to filter your data rows in Catalog. For a summary of each filter's compatibility with every data type, please see Search and view compatibility.
Attribute | Description |
---|---|
Annotation | Find data rows with labels that contain or do not have certain counts of annotations |
Batch | Find data rows that belong to a particular batch |
Data row | Filter based on global key, data row ID, created at, and last activity at |
Dataset | Find data rows that belong to a particular dataset |
Find text | Find data rows that contain a particular keyword |
Issue | Find labelled data rows that have Issue |
Label Actions | Find data rows that have a label(s) or haven't been labeled yet |
Media attributes | Find data rows based on their media type (e.g., image, video, text) or other attributes computed upon upload (e.g., video duration, height, width) |
Metadata | Find data rows that contain a certain metadata field and/or value |
Model | Find data rows used in Foundry |
Model prediction | Find data rows that have Foundry prediction(s) |
Natural language search | Filter based on natural language (e.g., photos of birds in grass fields) |
Project | Find data rows that are associated with a specific labeling project |
Similarity | Automatically surface data rows that are more or less similar to selected data rows |
Benchmark agreement | Find data rows with certain benchmark agreement metrics by label or feature |
Consensus agreement | Find data rows with certain consensus agreement metrics by label or feature |
How filters work
Think of creating a filter like constructing a pyramid with layers of logical sequence. Each layer is an AND
operation. Within a layer, you can use OR
operations. Each filter provides a count of data rows that match the filter. Only non-zero counts of instances of an attribute are available for selection and provided as a possible selection.
Labelbox search and curation capabilities allow for unmatched flexibility, scale, performance, and automation.
Flexibility
Labelbox search is uniquely flexible. Users can combine all kinds of filters in any arbitrary way. You can do both structured searches and unstructured searches at the same time.
- Structured searches include filters on metadata, annotations, metrics, confidence, datasets, projects, and more.
- Unstructured searches include similarity search, natural language search, and text search.
Combine different kinds of search results for even more powerful data curation. Training datasets that are carefully visualized, curated, and debugged are the most successful for increasing model performance.
Data Search
Catalog offers the flexibility to search for keywords without specifying a filter. You can directly type in the search bar and it will display suitable options:
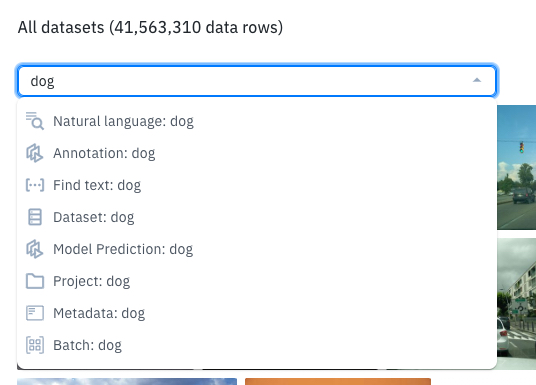
Typing "dog" here suggests this type of filters
Scale
Labelbox search operates at a scale of 100+ million data points. Building an in-house data catalog that scales to hundreds of millions of data points and provides instant results instantaneously in just one click is difficult for even the most advanced machine learning teams.
Performance
Labelbox strives to deliver high-performing search capabilities. Search queries take less than 15 seconds to return results, even on hundreds of millions of data points.
Visual search
Labelbox search results are visual. This makes it easy to refine and iterate on a search. Given the results from the previous search, you can easily add, remove, or edit filters. With Labelbox, non-technical teams have the most powerful data exploration and curation at their fingertips.
For a summary of data visualization capabilities for every data type, please see Search and view compatibility.
Automatic search
After populating filters in Catalog, you can save these filters as a slice of data. When you save a filter as a slice, you will not need to populate the same filters over and over again. Slices are dynamic; thus, any incoming data rows in your Catalog will appear in the relevant slices.
Read through the following resources to learn how to take action on the filtered data:
- Refine the similarity search
- Send filtered data rows to a labeling project as a batch
- Add metadata to the filtered data rows
Ascending or Descending
To gain a deeper understanding of your data, consider filtering based on the date of import, the latest activity on a data row, or metadata. This approach allows for a more dynamic comprehension of how your data is functioning and helps identify areas that may require additional resources.
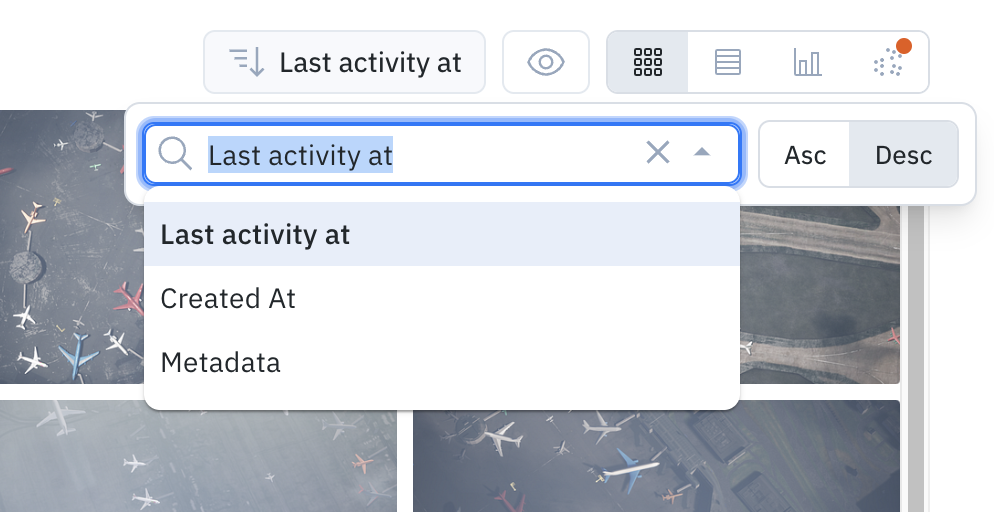
Collaborative search
Users can easily share search results with each other by sharing slice URLs.
Updated 10 months ago