Workflows
Create customizable, multi-step review and rework pipelines.
Workflows let you customize your review queue for greater efficiency and automation. A workflow consists of tasks. Each task serves a specific role in the review process and is fully configurable, allowing the flexibility in designing your review pipeline. For example, you can create a custom task to filter and review data rows with certain annotations or those labeled by a specific labeler or group.
If you have the admin or project lead role assigned, you can use the project Workflow tab to create, review, and maintain the review workflows. You can also set filtering criteria to control which data rows are sent to which review tasks.
Default workflow tasks
The default workflow has the following four tasks:
- Initial labeling task: reserved for all data rows that have been queued for labeling
- Initial review task: first review task for data rows with submitted labels
- Rework task: reserved for data rows that have been rejected. All data rows rejected at any of the intermediate review tasks are automatically sent to the Rework task without manual intervention.
- Done task: reserved for data rows that have either:
- Moved through their qualified tasks in the workflow
- Didn't qualify for any of the tasks
Among the default tasks, only the Initial review task allows you to change its name and add filtering criteria. Since the review process is iterative, workflows automatically update as data rows are labeled or rejected.
How data rows move through the workflow
A project workflow is similar to a conveyor belt, where data rows move along sequentially through the tasks like checkpoints. Here is how a data row moves through a workflow.
-
If a data row fits the filtering criteria for a review task, the data row will enter that review task. Then, there are two possible outcomes:
a. The data row gets an Approve review and goes on to the next task.
b. The data row gets a Reject review and gets directed to Rework (all rejected).
-
If a data row does not fit the criteria for a review task, it automatically gets passed to the next review task until it reaches a task that does fit the criteria.
-
Once a data row is modified/reworked in the Rework (all rejected) task, it goes through each task in the workflow that fits the criteria.
If a data row receives an Approve review and meets the criteria for both review tasks, the data row will go through the following tasks: Initial labeling task -> Labeler review -> Annotation review -> Done. If the data row receives a Reject review in either of the review tasks, it will move to the Rework task. Hence, a successful step-by-step flow is: Rework (all rejected) -> Labeler review -> Annotation review -> Done.
Create a new task
You can create custom review tasks in addition to the default Initial review task as part of your workflow:
- Click the Add new workflow task button and add:
- Task name: a high-level description of the review task
- Instructions: instructions on how to review data rows for this particular task
- Click + Add to select one or multiple Filters that determine whether data rows qualify for the review task.
Filter | Description |
---|---|
Labeled by | The user who labeled the data row |
Annotation | The label added to the data row |
Labeled at | The date range for when the label is added |
Consensus average | A range of average consensus scores to filter on each data row |
Dataset | The dataset where the data row comes from |
Issue category | The issue category of issues on the data row |
Batch | The batch where the data row is sent |
Metadata | The non-annotation metadata information on the data row |
Model prediction | The Foundry model predicted label of the data row |
Labeling time | The labeling time spent on the data row |
Review time | The review time spent on the data row |
Natural language | A natural language expression and confidence score range matching data rows with similar vector embeddings. |
Label feedback | The label feedback score range |
Sample probability | The likelihood of a data row being included in the task. For example, a sample probability of 40% means each data row that meets the other filters has a 40% chance of being included in the task. |
How Sample probability works with other filters
Sample probability is processed after all other filters. It's a probability, not a guarantee, so it's possible that none or only a few data rows are included for the task, even if many data rows meet the other filters.
Task limit
To view the maximum number of tasks per workflow, see limits.
Work on a task
- To see the data rows in a given task, click on the task name and click View data.
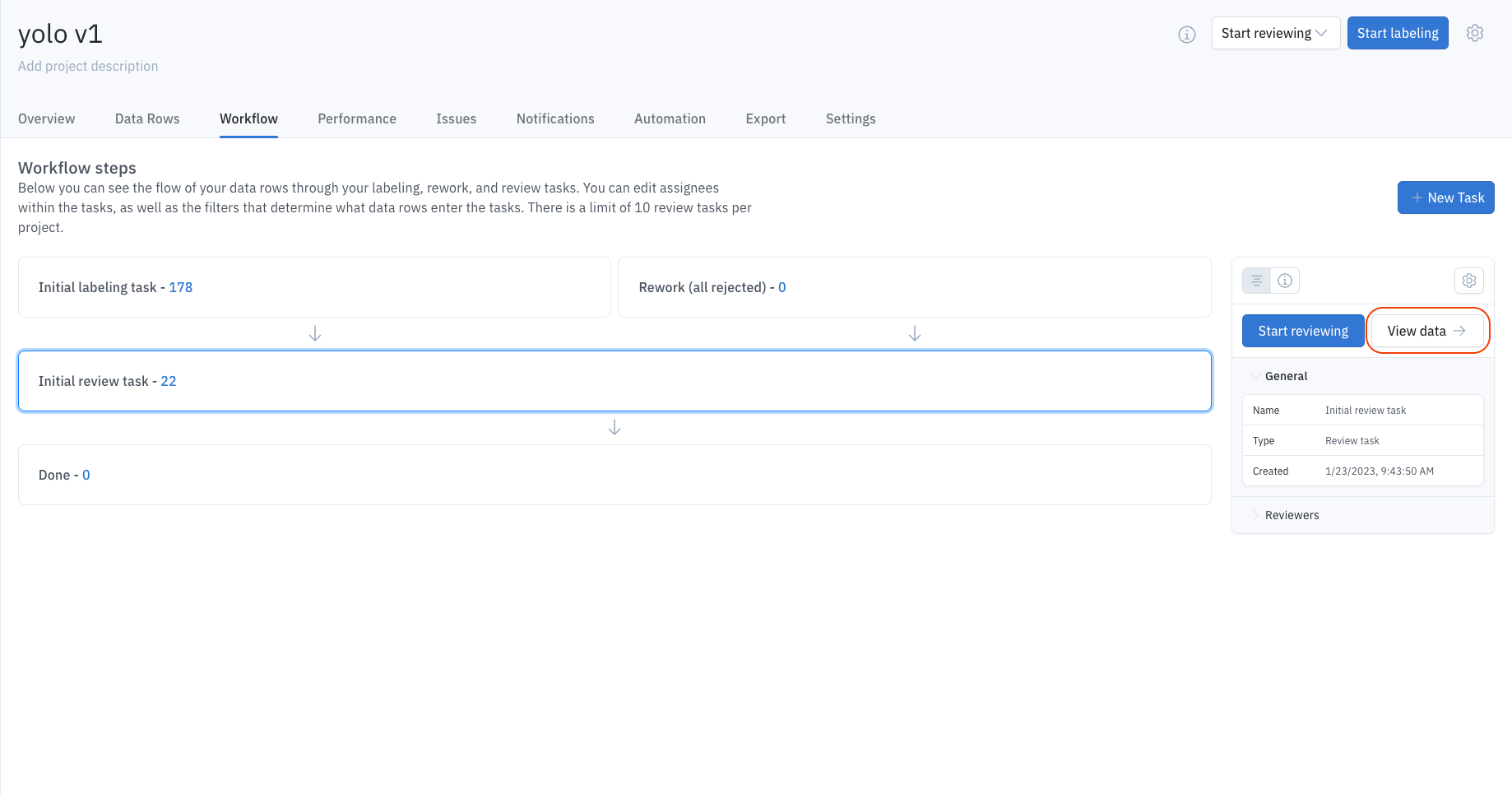
- To start working on a review task, click on the task and click Start reviewing in the task details to the right.
- This will open another browser where you can review assets with Approve and Decline reviews on a data row. Approved data rows will move to the next applicable review task, while declined data rows will move to Rework.
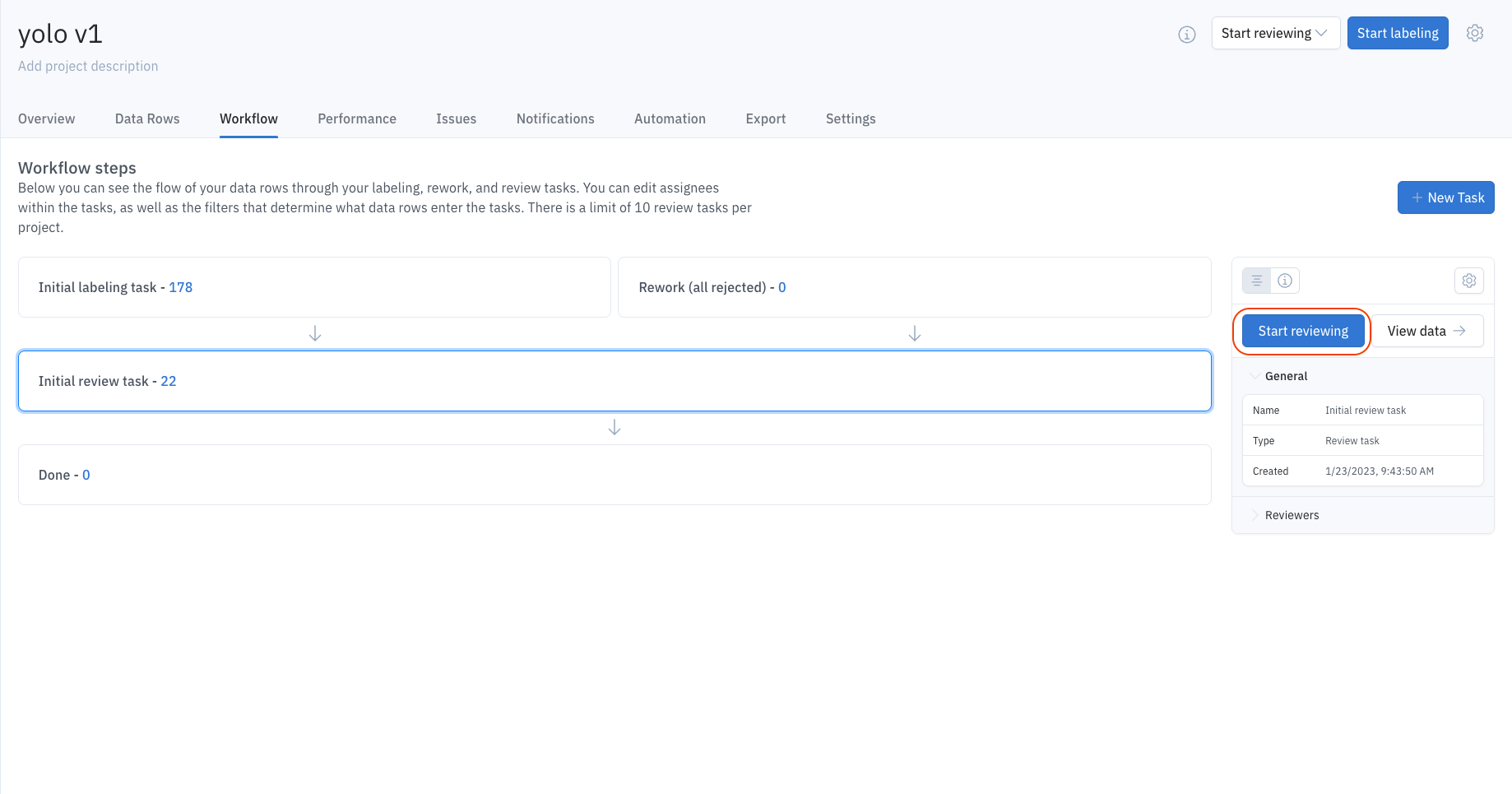
Configure and close tasks
Workflows are designed to be highly configurable. Configuring workflow tasks offers you the most flexibility to drive the efficiency of your QA process.
To modify any task, go to Edit task and modify the filter or add a new filter. You can also delete a filter by clicking the trash can icon next to the filter criteria.
To close a task (if you don’t need the task anymore), click on the gear icon and click Close task.
Condition to close a task
You can only close the last review task, and all data rows will be moved to Done section. Intermediate tasks can't be closed directly.
Reject & rework data rows
The rework flow is designed to automate the flow of specifying which data rows need to be fixed. The Move to task button allows you the flexibility to maintain a structured workflow while being able to manually move data rows to another task, if necessary. Moving data rows to different tasks in a single bulk action is also covered in the audit logs.
Send a single data row to rework
To reject a single data row and send it to rework:
- Select the task on the Workflow tab
- Click Start Reviewing
- Click Reject on the data rows you want to send to rework. All rejected data rows are automatically sent to the Rework task.
Bulk send data rows to rework
To bulk reject a set of data rows and send them to rework:
- Go to the Data rows tab
- Use the filters to specify the task the data rows are in
- Select a set of data rows (max 10,000 at a time)
- From the dropdown, select Move to task & select Rework
Labelers can rework data rows
Labelers (in addition to reviewers) can rework labels on any data rows sent to the Rework task, regardless of whether they created the label. The rework task offers the same functionality as the legacy delete and requeue workaround, which will be sunsetted.
Create an ad-hoc review task
In addition to the structured workflow, sometimes it can be helpful to conduct ad-hoc reviews to get a regular pulse check on the quality of the annotations from your project. However, all reviews must be done through workflows, so you must create another task and add it to your project's workflow.
Even with a structured workflow, this allows you to flexibly do ad-hoc reviews and keep track of them in an audit trail.
To create an ad-hoc task:
- Click on the New task button.
- Customize the filters, if any.
- Go to the Data rows tab.
- Select data rows in bulk and select Move to task.
- Go back to the Workflow tab.
- Click on the ad-hoc task you created and click Start Reviewing.
Moving through tasks with benchmarks
At any stage of the project, if a label on a data row is set as a benchmark, the data row (and thus any current and future labels made on the data row) will move to the Done task until the benchmark status is removed from the label.
If you are trying to review a data row with a benchmark label, remove the benchmark from the label and then proceed to move the data row to the appropriate task.
View audit logs
The audit logs allow admins to know the entire set of events that happened on a data row after it was labeled. Labelbox automatically creates this audit log for all data rows that enter the workflow. The purpose of the audit log is to help you understand the complete journey of each data row, especially when you need to investigate the review history of a particular data row or set of data rows.
To view the audit log for a data row:
- From the Workflow tab, click on a task. This will open another browser where you can see the data row.
- Click on the clock icon.
This will show the complete review history for a data row after it is labeled.
Updated 3 months ago